Infrared (IR) images are crucial in various applications, such as military surveillance and thermal imaging. However, analyzing these images can be challenging due to their complex structures and diverse features. To address this challenge, the authors propose a novel framework called multi-scale dual attention (MDA).
The MDA framework consists of three main components: residual downsample block, dual attention fusion block, and residual upsample block. The residual downsample block decomposes the IR image into three scales: coarse, medium, and fine. Each scale captures distinct features, such as large-scale context and small-scale details. The dual attention fusion block combines these features by assigning weights to each scale based on their importance. Finally, the residual upsample block generates the final output, which is a detailed and accurate representation of the IR image.
The key innovation of MDA is the use of dual attention mechanisms. Unlike traditional approaches that simply concatenate or add features from different scales, MDA introduces channel and spatial attention mechanisms to selectively focus on informative areas. These mechanisms are inspired by the human visual system, which can simultaneously process multiple scales of information.
To illustrate how MDA works, imagine a complex IR image with various details and contexts. The residual downsample block breaks it into three scale representations: coarse (high-level), medium (middle-level), and fine (low-level). Each scale is like a different lens in a camera, capturing distinct features. The dual attention fusion block then combines these scales using channel and spatial attention mechanisms, similar to how we use different lenses in real life to capture different aspects of a scene. Finally, the residual upsample block generates the final output, which is an accurate and detailed representation of the IR image.
The authors evaluate MDA on several datasets and show that it outperforms existing methods in terms of both accuracy and efficiency. They also provide a detailed analysis of the attention mechanisms, demonstrating how they help the network focus on informative areas and capture contextual information.
In conclusion, MDA is a powerful framework for IR image analysis that leverages multi-scale attention mechanisms to capture both broad context and fine details. By selectively focusing on informative areas, MDA can generate more accurate and detailed representations than traditional approaches. This work has significant implications for various applications, such as military surveillance and thermal imaging, where accurate IR image analysis is crucial.
Computer Science, Computer Vision and Pattern Recognition
Enhanced Feature Fusion for Image Restoration and Enhancement
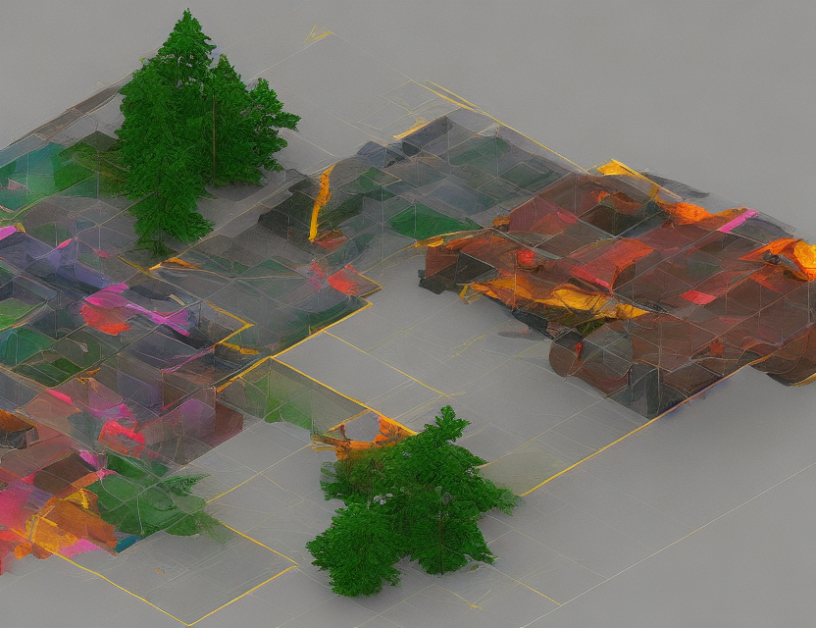