In this research paper, the authors aim to address the challenge of generating high-fidelity 3D models from a single sketch. The approach is based on disentanglement, which is similar to the "where" and "what" principles in generative models. By offering more visual clues, the model can generate a complete and accurate 3D model from a single sketch.
The authors explain that previous methods required a large dataset for evaluation, which is not always friendly for novice users. Other approaches use template primitives or retrieval-based approaches, but lack customizability. To overcome these limitations, the proposed method exploits human sketches to develop an intuitive and fast 3D modeling approach that generates high-fidelity models representing the creator’s intention.
The authors demonstrate the effectiveness of their approach by outperforming existing state-of-the-art methods in most categories, even without domain adaptation (DA). The proposed method has the potential to revolutionize 3D modeling by providing an easy-to-use and efficient way to generate high-quality models from a single sketch.
Analogy: Imagine you are trying to build a complex Lego castle using only a few scattered instructions. It would be challenging, right? Now imagine if you had a magical tool that could turn those scattered instructions into a complete and accurate castle model, just by looking at it once. That’s what the proposed method does – it takes a single sketch as input and generates a high-fidelity 3D model that represents the creator’s intention.
In summary, the authors propose a novel approach to generate high-quality 3D models from a single sketch using disentanglement. The approach is designed to be intuitive, fast, and efficient, making it accessible to novice users. The proposed method has the potential to revolutionize the field of 3D modeling by providing an easy-to-use tool for generating high-quality models from a single sketch.
Enhancing Sketch Recognition with Visual Clues
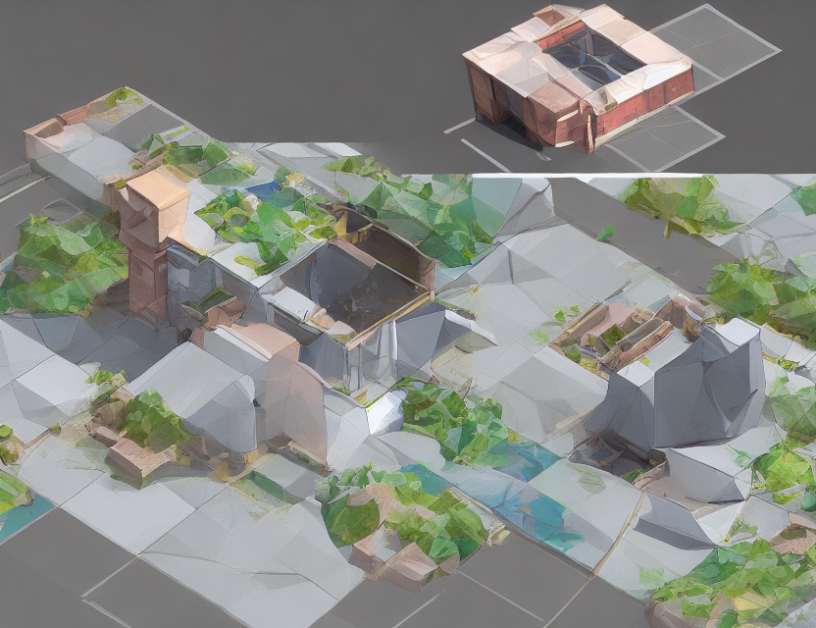