In this article, we explore the concept of knowledge-driven approaches in driving scenario understanding. We delve into the evolutionary trends in AI and examine how these approaches differ from traditional rule-based and data-driven methods.
Knowledge-driven approaches rely on semantic understanding to represent scenes and events in the real world. These methods aim to concretize and generalize human experiences, allowing for improved reasoning and decision-making abilities. Unlike rule-driven paradigms, which rely on meticulous logical reasoning or empirical validation using manually crafted rules, knowledge-driven approaches use internal memory to store and update driving experiences.
The internal memory constructs a dynamic perception of the environment, enabling the system to retain and update its understanding of the surroundings. Historical driving experiences and knowledge are archived in this memory, which is crucial for addressing similar scenarios. Long-term memory fine-tunes the foundation model, allowing for rapid reasoning, while short-term memory preserves recent and unfamiliar scenarios, ensuring swift adaptation to diverse environments.
In conclusion, knowledge-driven approaches represent a significant advancement in driving scenario understanding. By leveraging internal memory, these methods enable AI systems to adapt to complex scenarios with improved decision-making abilities. While traditional rule-based and data-driven methods have their limitations, knowledge-driven approaches offer a promising solution for enhancing autonomous driving capabilities.
Evaluating Image Captions with Diversity: A New Metric
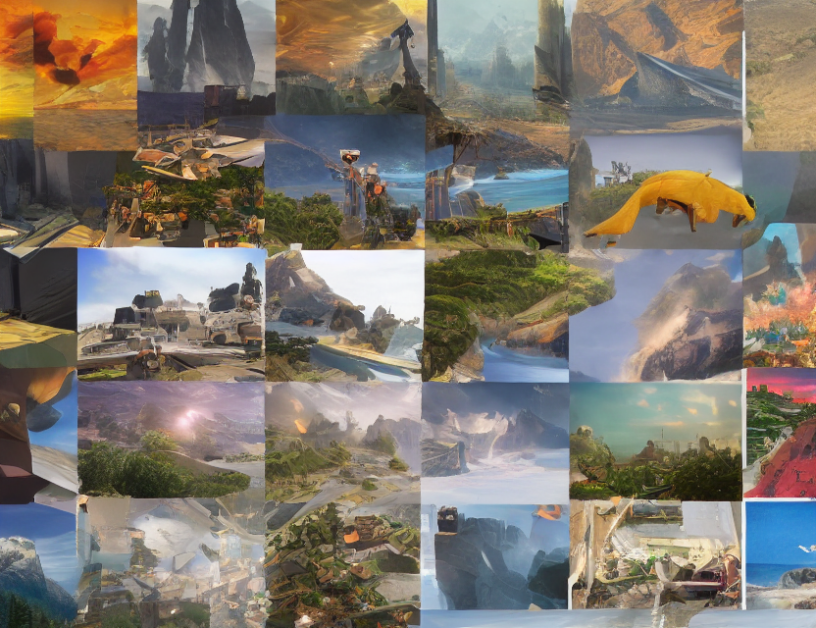