In this article, researchers present a groundbreaking approach to generating high-quality 3D models from textual prompts. This innovative method, called Point-e, leverages the power of neural networks to create 3D point clouds that match the complexity and details described in the given text. The authors build upon previous works that utilized 2D priors or diffusion models but improve upon them by introducing a new architecture that optimizes the underlying 3D representations.
To understand how Point-e works, imagine a text editor with a feature called "Text-to-Image." Instead of simply generating an image based on the entered text, Point-e creates a detailed and realistic 3D model that can be rotated, zoomed, and examined from any angle. This is achieved by using a combination of neural networks and optimization techniques to refine the generated 3D model until it matches the described textual prompt.
The authors evaluate their approach on several challenging categories from ShapeNet and Objaverse datasets, demonstrating impressive results that rival those of state-of-the-art methods. They showcase the capability of their framework to capture fine details and produce rich and diverse textures by merely modulating the textual prompts.
In summary, Point-e represents a significant breakthrough in the field of computer vision and generative models. By leveraging the power of textual descriptions, this method has the potential to revolutionize various applications, such as 3D modeling, computer-aided design (CAD), virtual reality (VR), and video games. With its impressive performance and versatility, Point-e is poised to transform the way we interact with 3D models in the future.
Computer Science, Computer Vision and Pattern Recognition
Generating Conditional 3D Implicit Functions via Shap-e
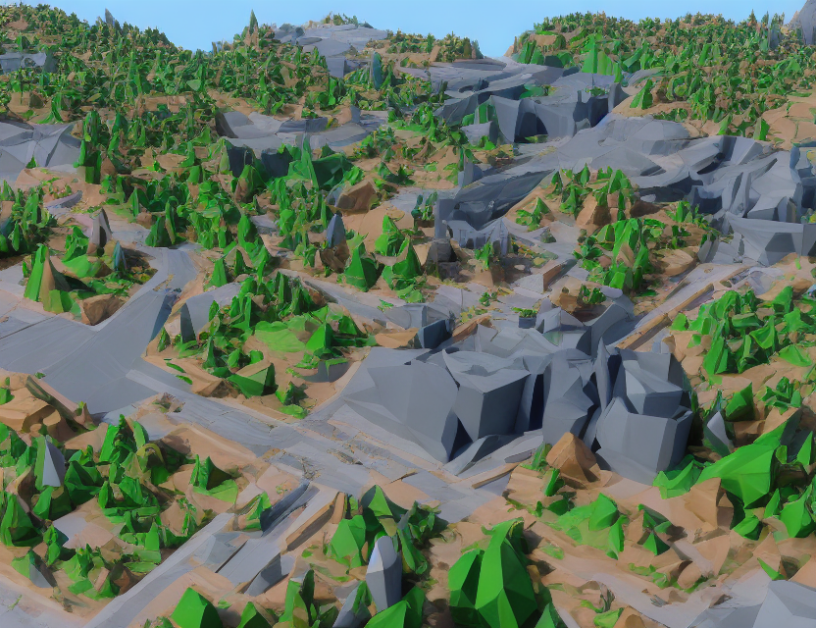