Contact Graphs are a crucial component in humanoid robotics, enabling robots to interact with their environment and perform various tasks. The article delves into the concept of Contact Graphs, exploring how they are used to model the contact between objects in a scene, including the hand, ball, and table. The authors propose a novel approach called Conditioned Grasping with Real-world Contact (CGR), which incorporates real-world contact information to improve grasping performance.
The article explains that traditional grasping methods rely on predefined grasps, which can result in suboptimal performance when encountering complex objects or unexpected contact scenarios. CGR addresses this issue by conditioning the grasps based on real-world contact information, enabling the humanoid robot to adapt to novel situations and learn from experience.
The authors demonstrate the effectiveness of CGR through experiments involving a humanoid robot interacting with various objects, including a ball and a table. The results show that CGR outperforms traditional grasping methods in terms of accuracy and versatility, illustrating its potential to enhance the robot’s ability to adapt to changing environments.
The article also discusses the importance of considering contact with multiple objects and the role of tables in maintaining balance during grasping tasks. The authors emphasize that CGR can address these issues by involving the table in the contact graph, ensuring a more comprehensive understanding of the robot’s surroundings.
In summary, the article provides a detailed analysis of Contact Graphs and introduces a groundbreaking approach called CGR, which leverages real-world contact information to improve grasping performance in humanoid robotics. The proposed method has the potential to revolutionize the field by enabling robots to adapt to novel situations and interact more effectively with their environment.
Computer Science, Computer Vision and Pattern Recognition
Generating Human Motion From Textual Descriptions: A Survey
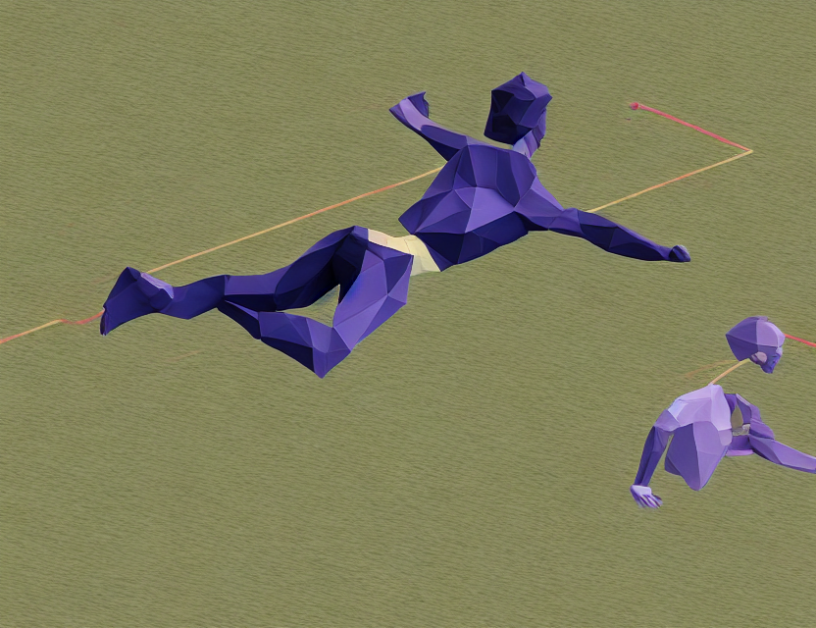