In this research paper, the authors aim to improve the efficiency of a text-to-image synthesis model by distilling the generation ability of an existing model called SDXL. To do this, they conduct an in-depth analysis of SDXL’s denoising U-Net, which has the most number of parameters and computational cost among all the layers in the model. They find that most of the parameters are concentrated at the lowest feature level due to the large number of transformer blocks.
To create a more efficient U-Net, the authors reduce SDXL’s U-Net by up to 69% while preserving its generation ability. They also investigate how to effectively distill SDXL as a teacher model and identify four essential factors for feature-level knowledge distillation. These factors are self-attention features, which are crucial for capturing semantic affinities and the overall structure of images.
The authors find that distilling self-attention features achieves the most performance gain compared to other types of features. They also emphasize that self-attention plays a vital role in capturing semantic affinities and the overall structure of images, making it essential for the distillation process.
In summary, the authors of this paper aim to improve the efficiency of a text-to-image synthesis model by distilling the generation ability of an existing model called SDXL. They conduct an in-depth analysis of SDXL’s denoising U-Net and identify key factors for feature-level knowledge distillation. The authors find that distilling self-attention features achieves the most performance gain, emphasizing the crucial role of self-attention in capturing semantic affinities and the overall structure of images.
Computer Science, Computer Vision and Pattern Recognition
Improving Image Generation with Better Captions
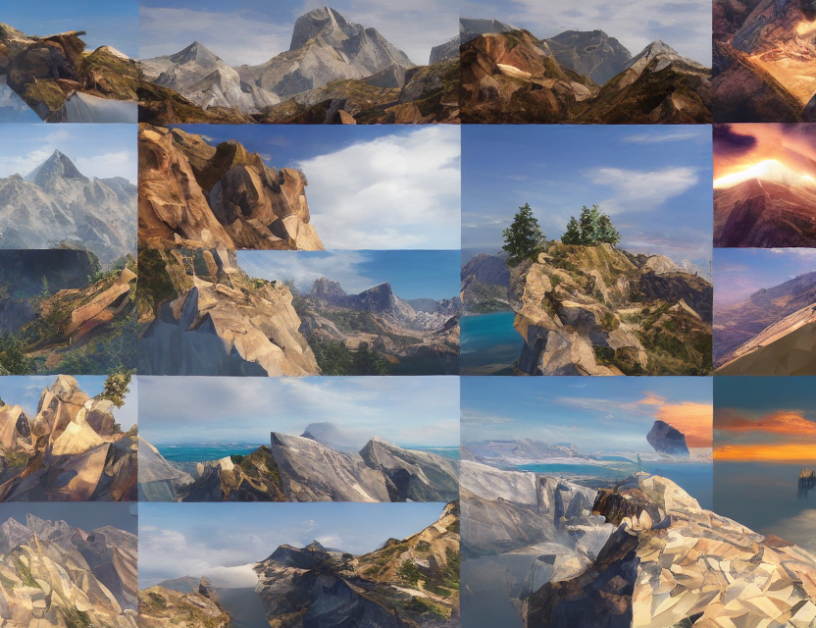