In this article, we propose a new approach to semantic segmentation, which is a process of assigning labels to each pixel in an image to identify objects and their boundaries. Our method leverages a small number of human-labelled samples and combines them with automatically generated pseudo labels to improve model performance without oversimplifying the process.
To begin with, we discuss existing methods for semantic segmentation, which typically require a large number of labelled images. However, obtaining such labels can be time-consuming and expensive. Our approach addresses this challenge by using a sparse set of human-labelled samples and combining them with pseudo labels generated from an uncertainty-aware semantic map.
The core idea is to select a small number of informative pixels for human labelling and combine them with automatically generated pseudo labels to create a semi-supervised learning framework. By doing so, we can reduce the amount of human labour required for labeling while preserving similar performance and outperforming purely self-supervised approaches.
To accomplish this, we propose a new pixel selection procedure that balances the informativeness and diversity of pixels. We then compute the pseudo labels from the model predictions of unlabelled data using an uncertainty-aware approach. By combining human labels with pseudo labels, our method can significantly reduce the amount of labelled data required while maintaining comparable performance to fully supervised methods.
Our proposed method has several advantages over existing approaches. Firstly, it reduces the amount of human labour required for labeling, making it more practical and efficient. Secondly, it improves the performance of the model by combining human labels with pseudo labels generated from an uncertainty-aware semantic map. Finally, our approach can be easily integrated with new components such as novel pixel selection methods or automatic pseudo label generation techniques, which could further improve the performance of semi-supervised robotic active learning.
In summary, our article presents a novel approach to semantic segmentation that leverages a small number of human-labelled samples and combines them with automatically generated pseudo labels to improve model performance without oversimplifying the process. By reducing the amount of human labour required for labeling and improving the performance of the model, our method has the potential to make semantic segmentation more practical and efficient in real-world applications.
Leveraging Uncertainty-Aware Pseudo Labels for Sparse Semantic Segmentation
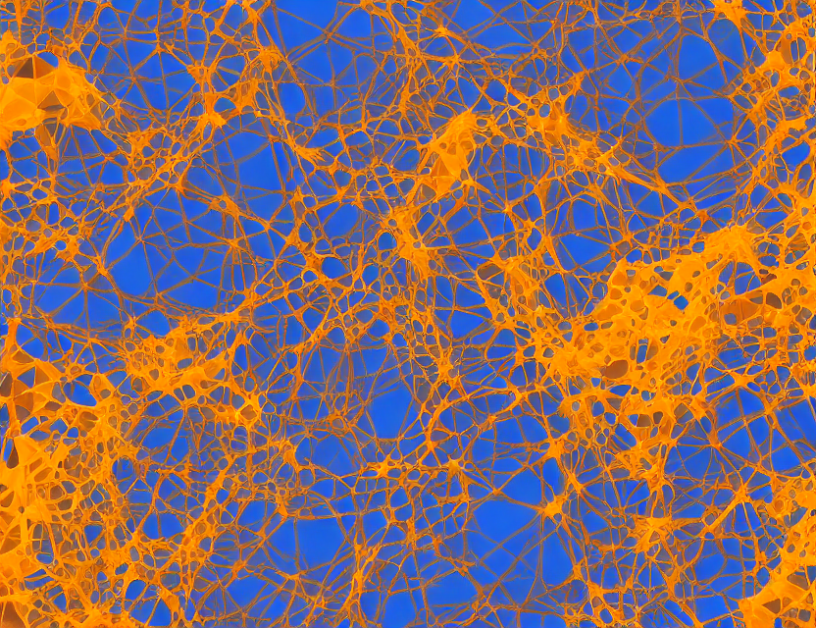