In this article, the authors propose a novel approach to generating high-quality images from a single input photo using a combination of text and diffusion models. The key idea is to use a text encoder to generate a contextual representation of the input image, which is then used as input to a diffusion model that progressively refines the generated image. This process allows for controllable and efficient image synthesis, enabling the creation of novel views from a single input photo.
The authors build upon previous research in text-guided image synthesis, where the focus was on generating images based solely on the input text. In contrast, their approach leverages both the text context and the original image to generate high-quality images that are consistent with the provided text description. This is achieved through a novel attention mechanism that combines the text and image representations, allowing the model to selectively focus on different regions of the input image based on the given text context.
To train their model, the authors use a combination of reconstruction loss (to ensure that the generated images are similar to the original input) and a adversarial loss function that encourages the generated images to be consistent with the provided text context. This leads to improved synthesis quality and increased controllability over the generated images.
The proposed model is evaluated on several benchmark datasets, demonstrating its effectiveness in generating high-quality novel views from a single input photo. The authors also provide an ablation study to analyze the contribution of different components in their approach, further highlighting the efficacy of their method.
In summary, this article presents a novel approach to text-guided image synthesis that leverages both text and diffusion models for efficient and controllable image generation from a single input photo. By combining the strengths of these two approaches, the authors are able to generate high-quality images that are consistent with the provided text context, making this method highly versatile and practical for a wide range of applications.
Computer Science, Computer Vision and Pattern Recognition
Text-Guided Diffusion Models for Image Generation and Editing
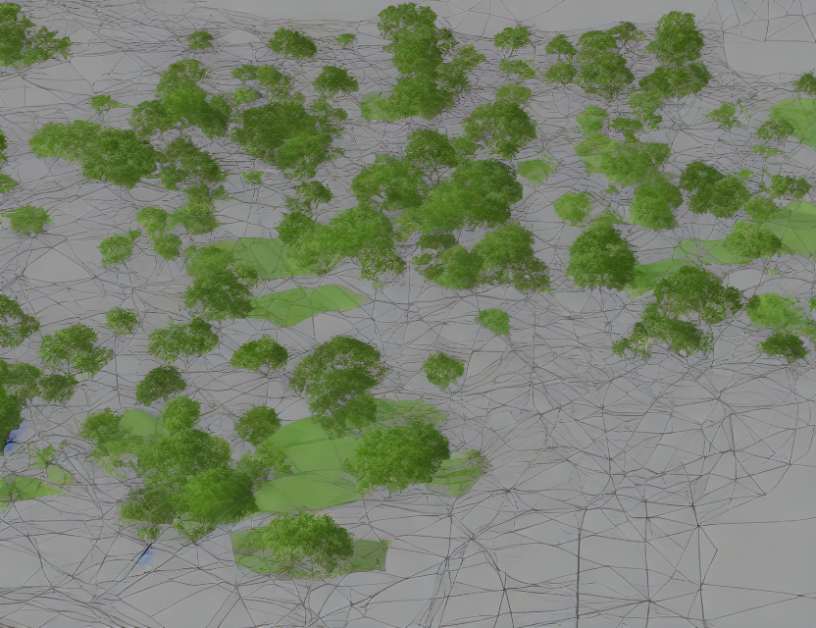