The article discusses the challenges and opportunities in text-to-video finetuning, a technique used to generate videos based on textual descriptions. The authors present several recent approaches that have shown promising results in this field, including textual inversion, custom diffusion, and Dreambooth. They also outline some of the limitations and challenges associated with these methods, such as the need for diverse and high-quality training data, the potential for overfitting, and the difficulty in controlling the generated video’s content and quality.
To address these challenges, the authors propose several ablation studies that aim to improve the performance and adaptability of text-to-video finetuning models. These studies include exploring different spatial and temporal layers, using various sampling strategies, and incorporating regularization techniques to prevent overfitting. The authors also introduce a novel sampling strategy that utilizes both uniform and coarse-noise samples to improve the quality and diversity of the generated videos.
The authors conclude that while text-to-video finetuning has made significant progress in recent years, there are still several challenges and limitations that need to be addressed to create more realistic and controllable video generation models. They suggest that further research is needed to develop more sophisticated and adaptive models that can generate high-quality videos based on textual descriptions.
In summary, the article discusses the current state of text-to-video finetuning, including recent approaches and challenges, and proposes several ablation studies to improve the performance and adaptability of these models. The authors emphasize the need for further research to develop more advanced and controllable video generation models based on textual descriptions.
Computer Science, Computer Vision and Pattern Recognition
Text-to-Image Synthesis: Advancements and Applications
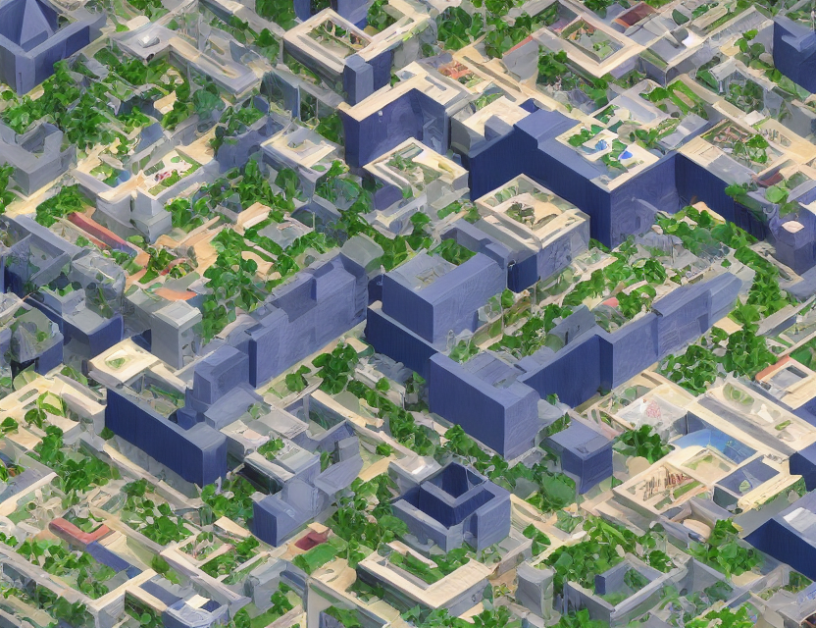