In this review, we will delve into the concept of cooperation in Multi-Agent Learning (MAL) environments. MAL is an emerging field that involves multiple agents learning and interacting with each other to achieve a common goal. Cooperation is essential in MAL as it allows agents to work together towards a shared objective while also considering their own self-interest.
The article highlights the importance of reputation and norms in cooperation. Reputation refers to an agent’s past actions and outcomes, which can influence its future behavior and decision-making. Norms, on the other hand, are collective patterns of behavior that are supported by shared sanctioning practices among agents. In MAL environments, norms can play a crucial role in promoting cooperation by providing a framework for agents to follow and expectations for their behavior.
The article also discusses how humans use their reputation within a group as motivation to cooperate. This idea is reflected in MAL environments through the use of intrinsic rewards, such as a sense of belonging or social approval, which can motivate agents to cooperate and follow norms.
Another line of research explores the role of centralized control in MAL environments. While centralized control can provide a convenient solution for gathering data and designing policies, it is often not practical or feasible in real-world applications. Instead, decentralized approaches that rely on distributed communication and decision-making can be more effective and efficient in promoting cooperation among agents.
In summary, this review highlights the significance of reputation, norms, and centralized control in MAL environments, as well as the challenges and opportunities presented by these concepts. By understanding these factors, researchers and developers can create more effective and sustainable MAL systems that promote cooperation and collaboration among agents.
Computer Science, Multiagent Systems
Cooperation in MAL: A Review of Algorithms and Strategies
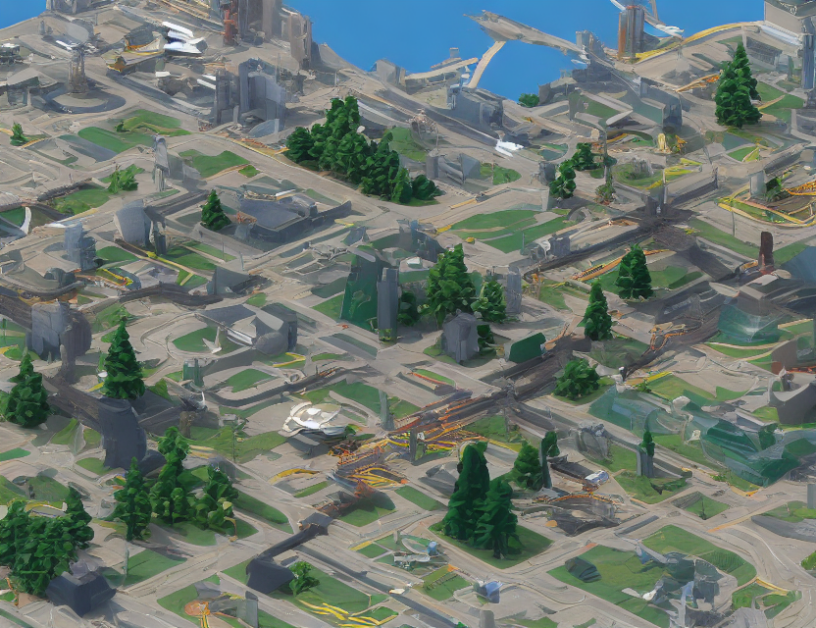