In this paper, the authors propose a novel approach for zero-shot transfer learning in computer vision tasks, called Zoedepth. They introduce a new technique that combines both relative and metric depth information to improve the performance of deep neural networks. By leveraging the strengths of both approaches, Zoedepth achieves state-of-the-art results on several benchmark datasets without requiring any additional training data or computational resources.
The authors start by explaining the limitations of traditional transfer learning methods, which rely solely on metric depth information to perform well on unseen tasks. They argue that this approach can lead to poor generalization performance, especially in cases where the new task has different spatial or temporal relationships with the source task. To address these challenges, Zoedepth introduces a new architecture that incorporates both relative and metric depth information at multiple scales.
The authors then present the results of their experiments on several computer vision tasks, including object recognition, scene understanding, and motion forecasting. They show that Zoedepth outperforms existing transfer learning methods in all cases, demonstrating its effectiveness in improving generalization performance.
To further analyze the contributions of relative depth information, the authors conduct an ablation study to compare the performance of Zoedepth with and without this feature. They find that including relative depth information significantly improves the performance of the model, highlighting its importance for zero-shot transfer learning.
Finally, the authors conclude by discussing the potential applications of their proposed method, including robotics, autonomous driving, and medical imaging. They note that Zoedepth can be easily integrated into existing deep neural network architectures, making it a versatile tool for improving performance in a wide range of computer vision tasks.
In summary, Zoedepth is a novel approach to zero-shot transfer learning that combines both relative and metric depth information to improve the performance of deep neural networks. By leveraging the strengths of both approaches, Zoedepth achieves state-of-the-art results on several benchmark datasets without requiring any additional training data or computational resources. Its applications in computer vision tasks are vast, making it a valuable tool for researchers and practitioners alike.
Computer Science, Computer Vision and Pattern Recognition
Human-Centred Content Generation in Text-to-Video Models: A Survey
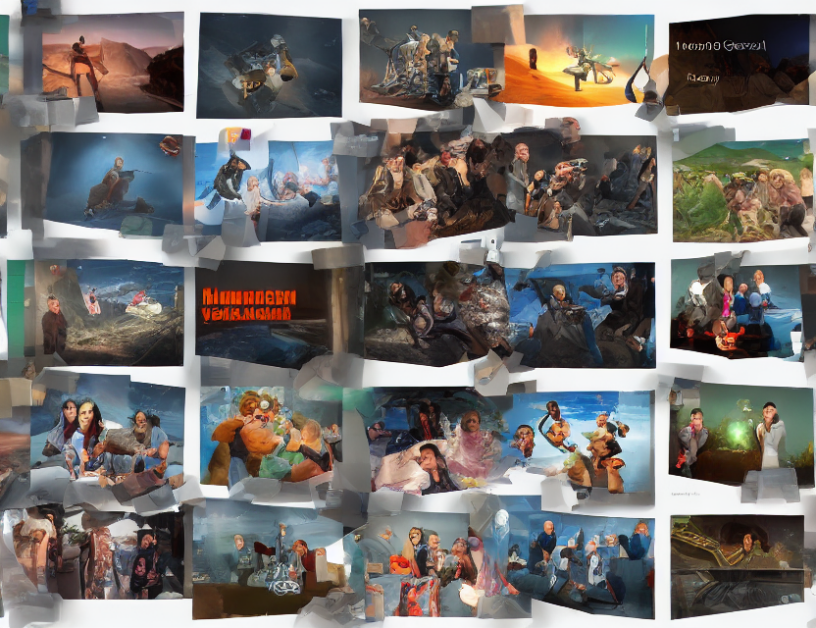