In this article, we will delve into the exciting field of text-to-3D synthesis, where AI models generate 3D objects and scenes from textual descriptions. The advent of large-scale vision-text datasets and powerful vision-language models has fueled significant progress in this area. Researchers have focused on single object synthesis, optimizing differentiable 3D representations through a loss signal derived from the denoising of rendered views. While these methods produce impressive results for object-centric scenes, they struggle to generalize to complex large-scale scenes.
To address this challenge, we will explore two notable approaches: SceneScape [15] and Text2Room [20]. SceneScape leverages off-the-shelf 3D data sources to generate text-driven consistent scene generation, while Text2Room focuses on extracting textured 3D meshes from 2D text-to-image models. Both methods aim to provide a seamless integration of textual descriptions and 3D visualization.
To demystify complex concepts, let’s consider an analogy: generating 3D objects is like building LEGO blocks. Just as we can use verbal instructions (text) to construct specific LEGO creations, AI models can utilize textual descriptions to generate detailed 3D structures. However, unlike LEGO blocks, which have predefined shapes and structures, 3D objects can take on an array of forms and dimensions, making their generation more challenging.
The article highlights the importance of large-scale vision-text datasets and powerful vision-language models in driving progress in text-to-3D synthesis. These models enable AI to learn and optimize differentiable 3D representations through a loss signal derived from the denoising of rendered views. While these methods have shown impressive results for object-centric scenes, they struggle to generalize to complex large-scale scenes.
To overcome this limitation, researchers have proposed two innovative approaches: SceneScape [15] and Text2Room [20]. SceneScape leverages off-the-shelf 3D data sources to generate text-driven consistent scene generation, while Text2Room focuses on extracting textured 3D meshes from 2D text-to-image models. Both methods aim to provide a seamless integration of textual descriptions and 3D visualization.
In conclusion, the article provides an in-depth exploration of text-to-3D synthesis, highlighting the challenges associated with generating complex large-scale scenes. By examining two notable approaches, SceneScape [15] and Text2Room [20], we gain a deeper understanding of how AI models can leverage textual descriptions to generate detailed 3D structures. This exciting field has enormous potential for applications in various industries, from entertainment to architecture, and it will be fascinating to witness the further developments in this area.
Computer Science, Computer Vision and Pattern Recognition
Image Synthesis and Text-Driven Scene Generation: A Review of Recent Approaches
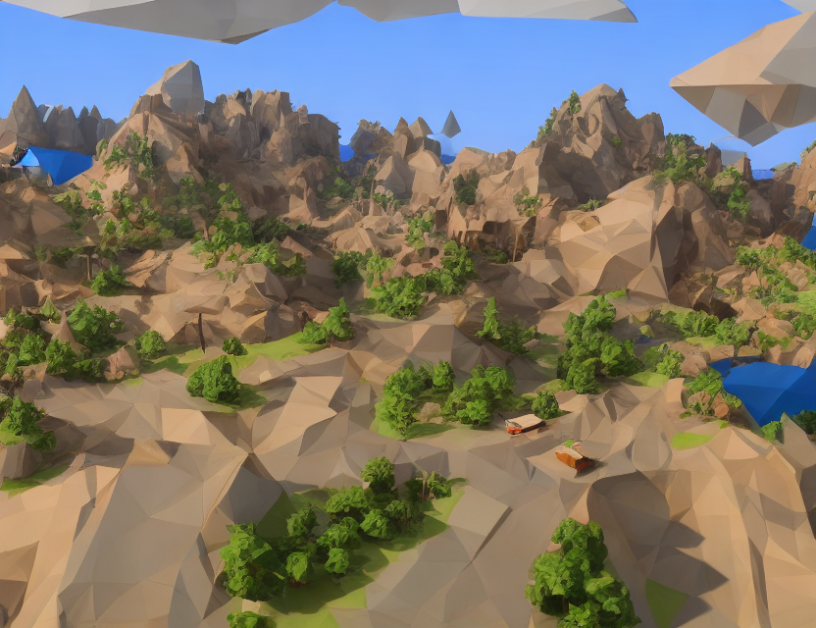