In this article, we explore the concept of multi-agent data fusion in decentralized robotics systems. We begin by discussing the benefits and limitations of this approach, which involves combining data from multiple sensors or agents to improve overall performance. We then present various fusion algorithms used in previous research, along with illustrations of their network topologies.
To understand multi-agent fusion, imagine a group of robots working together to complete a task. Each robot has its own sensor suite, providing different perspectives on the environment. By sharing this data, the robots can create a more accurate and complete picture of their surroundings. However, integrating data from multiple sources can be challenging, as it requires balancing the need for shared information with individual agent goals.
There are several fusion algorithms used in decentralized robotics, including Fusion at Perception, which involves sharing raw perception data between agents. This approach can lead to accurate results due to the richness of shared data, but it may not always be possible due to limited resources. Other fusion algorithms include covariance intersection and general decentralized data fusion with covariance intersection.
In conclusion, multi-agent data fusion is a powerful technique for improving robotic performance in decentralized systems. By combining data from multiple sources, robots can create a more accurate and complete picture of their surroundings. While there are challenges to integrating this data, various fusion algorithms have been developed to address these issues. As technology continues to advance, we can expect to see even more innovative solutions in the field of decentralized robotics.
Innovations in Autonomous Vehicle Datasets: Generating High-Quality MS/MA Datasets from Simulator Data
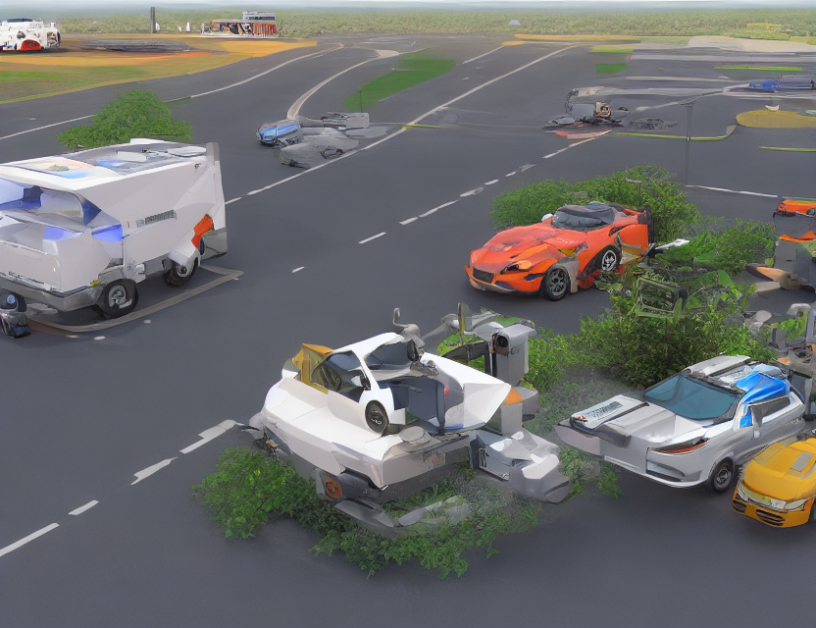