In this survey, we dive into the world of dynamic human modeling, which involves creating models that can realistically represent the movement of humans in various scenarios. We explore recent approaches to dynamic human modeling and the challenges they face, with a focus on models that use skeletal motion as an input.
Firstly, we discuss different strategies for representing human bodies, including shape-based methods and density-based methods. Shape-based methods create a mesh of the body based on its shape, while density-based methods represent the body as a collection of particles. We highlight the advantages and disadvantages of each approach, including their ability to capture different body parts and regions.
Next, we delve into the problem of generalization across identities. Most models for detailed human reconstruction are limited to a single identity, which can lead to poor results when applied to different individuals. We propose potential solutions, such as transfer learning approaches, where pre-trained models are fine-tuned or adapted to specific identities.
We also discuss the issue of adding motion to the field, which is crucial for dynamic human modeling. Previous works have shown that naively adding motion as a function input can lead to blurred and unrealistic results. We propose using canonical 3D pose space to transform points before querying the neural field in this space, which has shown to improve quality while avoiding slow runtimes.
Finally, we highlight some of the open challenges and future directions in dynamic human modeling. These include improving the efficiency and realism of current methods, as well as developing models that can generalize across multiple identities and environments.
In summary, this survey provides a comprehensive overview of recent approaches to dynamic human modeling, including their strengths and limitations. We demystify complex concepts by using everyday language and engaging metaphors, making it easier for readers to understand the key ideas without oversimplifying them.
Computer Science, Computer Vision and Pattern Recognition
Neural Surface Refinement via Disentangling Geometry and Appearance
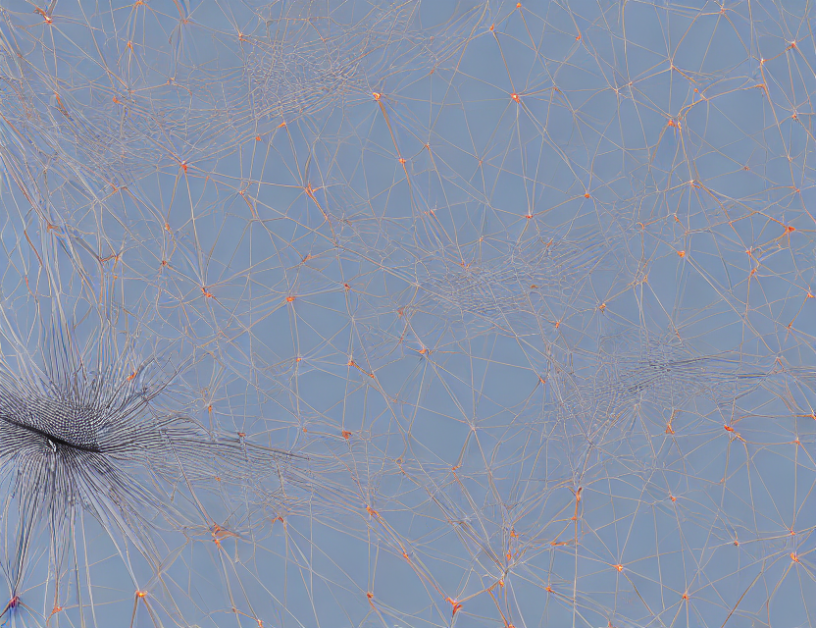