In this article, we explore the methodology used for conducting a systematic literature review in the field of few-shot learning. We delve into the specifics of selected digital libraries, search queries, inclusion and exclusion criteria, and the process of retrieving papers. Each paper is evaluated based on several criteria, with a focus on understanding their unique strengths and weaknesses without engaging in any discussion on task definition or used terminology.
We begin by explaining that, at this stage, we do not perform any analysis or discussion on task definitions or used terminologies but instead stick to the terms employed by the original authors to categorize these works according to their self-identified categories. Next, we present a table showing the total number of papers related to various settings and sub-queries.
The article then proceeds to examine different models that have been proposed for few-shot learning, including text-based methods like OntoPrompt [134] and CogKR [31], which leverages both text-based and ontology-based representations, as well as neural process-based approaches such as NP-FKGC [154] and RawNP [154]. These models aim to address issues like out-of-distribution, overfitting, and uncertainty in predictions by incorporating techniques like summary reasoning modules or stochastic manifold encoders.
We then discuss the importance of entity encoding and subgraph enclosing, highlighting their significance in capturing complex few-shot relationships. Finally, we conclude by emphasizing that rule-based methods can also be employed to tackle these challenges effectively.
Throughout the article, we strive to use everyday language and engaging metaphors or analogies to demystify complex concepts without oversimplifying them. Our goal is to provide a concise yet comprehensive summary of the article that captures its essence while maintaining accuracy and readability.
Artificial Intelligence, Computer Science
Systematic Survey of Digital Libraries for Emerging Entities
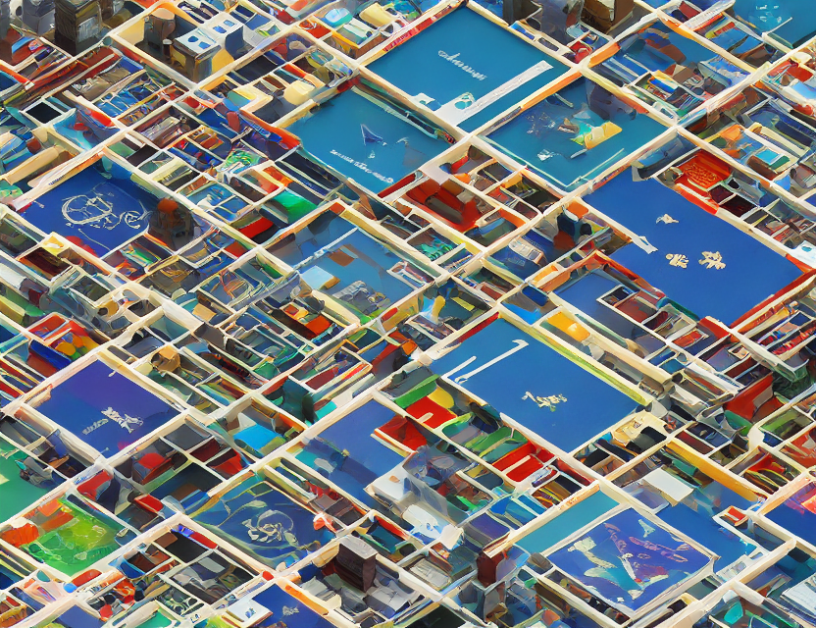