In this article, the authors explore the potential of coevolutionary dynamics to bridge the gap between artificial intelligence (AI) and natural evolution. They argue that traditional evolutionary algorithms are limited in their ability to capture the open-ended creativity inherent in natural evolution. To address this issue, they propose using coevolutionary dynamics, where populations evolve in response to each other to generate more innovative and adaptive solutions.
The authors begin by highlighting the diversity and complexity of life on Earth, which are testaments to the evolutionary process’s creative and adaptive capabilities. They note, however, that modern implementations of evolutionary algorithms often fall short in capturing this open-ended creativity. To overcome this limitation, they propose using coevolutionary dynamics, where populations evolve in response to each other to generate more innovative and adaptive solutions.
The authors then delve into the details of their proposal, explaining how agents can be evolved in response to changing environments. They show how this approach can lead to more open-ended and innovative evolutionary outcomes, embodied in the emergence of new adaptive strategies. The authors also discuss the importance of multi-objective reward systems in enabling diverse agents to succeed in various ways, fostering a cycle of continuous innovation and adaptation.
Throughout the article, the authors use engaging analogies and metaphors to demystify complex concepts. For instance, they compare the process of coevolutionary dynamics to a "dance" between populations, where each population responds to the other’s movements to create a dynamic and adaptive environment. They also use the example of a "fitness landscape" to illustrate how populations can evolve in response to changing environments, leading to more innovative and adaptive solutions.
In conclusion, the authors argue that coevolutionary dynamics have the potential to bridge the gap between AI and natural evolution, enabling more open-ended and innovative evolutionary outcomes. By embracing this approach, they believe we can create more robust and adaptive AI systems that better embody the essence of open-ended evolution.
Artificial Intelligence, Computer Science
Unlocking Open-Ended Evolution: Leveraging Coevolution and Novelty Search
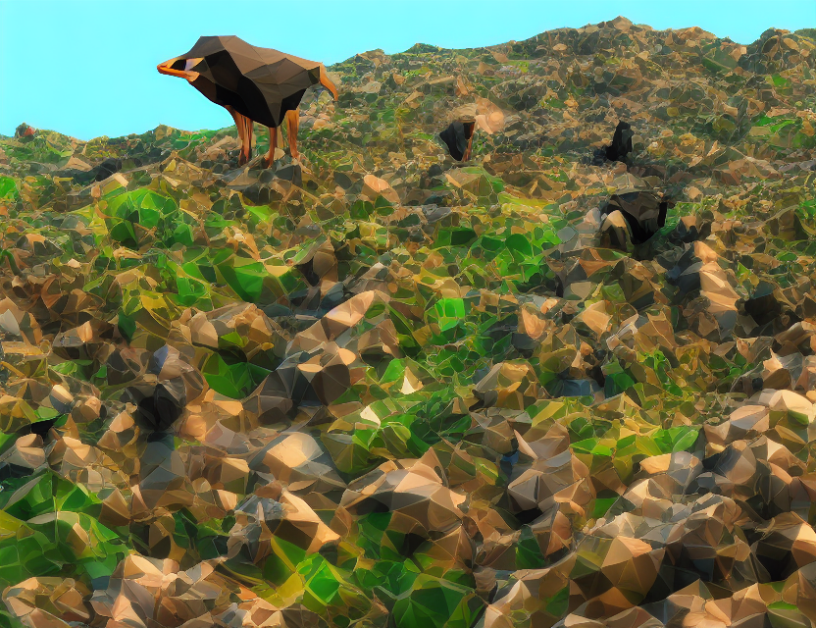