Causal inference is a crucial task in many fields, including economics, social sciences, and healthcare. However, real-world data often suffers from partial observability, where not all the variables of interest are directly observable. In such cases, accurate causal inference can be challenging due to the lost information about the main object of inference. This review aims to provide a comprehensive overview of the state-of-the-art methods for causal inference under partial observability, including their strengths, weaknesses, and applications.
The article begins by discussing the fundamental challenges in causal inference under partial observability, including the impact of noise and structural assumptions on the feasibility of inference. The authors then review several popular methods for dealing with partial observability, including feature-based approaches, Granger estimation, one-lag R1(n) estimator, NIG(R1(n))−NIG(R3(n)) estimator, and precision matrix or graphical Lasso for sparse regimes. Each method is described in detail, along with its strengths and weaknesses, to help readers understand their potential applications and limitations.
To illustrate the concepts, the authors use engaging analogies and metaphors to make complex ideas more accessible. For instance, they compare feature-based approaches to a puzzle solver trying to find the right pieces to fit together to infer causality, while Granger estimation is likened to a detective piecing together clues to solve a mystery. These analogies help readers connect with the concepts and visualize how each method works.
The review also highlights some of the challenges and open research directions in causal inference under partial observability, such as dealing with non-linear relationships and missing data mechanisms. The authors emphasize that these issues are critical for developing more accurate and robust causal inference methods, especially when working with complex systems or large datasets.
In summary, this comprehensive review provides a valuable resource for researchers and practitioners interested in causal inference under partial observability. By demystifying complex concepts and providing practical insights into the strengths and limitations of each method, it enables readers to make informed decisions about which approach to use in their own work. Whether you are a seasoned expert or just starting out, this review is an essential read for anyone tackling causal inference challenges in real-world applications.
Computer Science, Machine Learning
Inferring Structural Inference Methods for Nonlinear Dynamic Systems
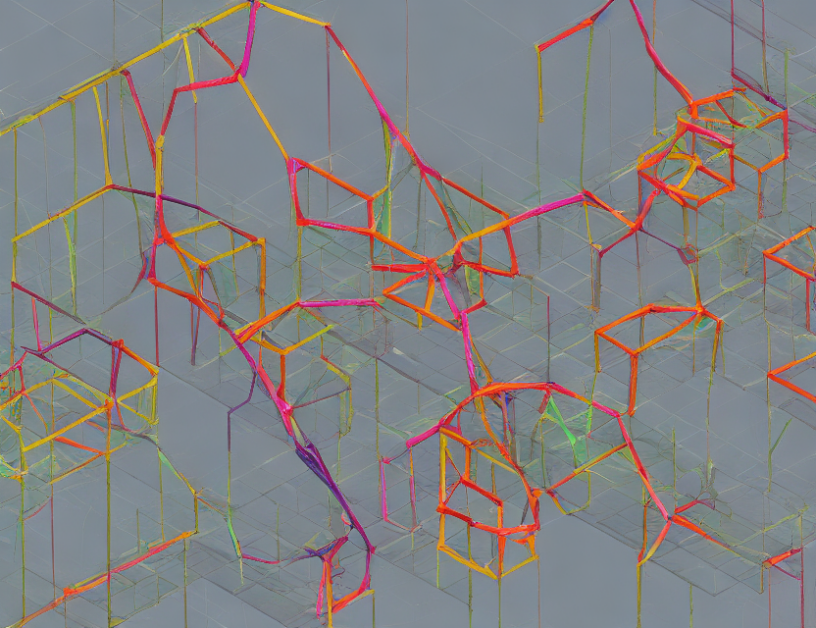