In this research paper, the authors propose a novel method for style transfer in images, which they call Content-Style Trade-Off (CSTO). The key innovation of CSTO is the introduction of a parameter, γ, that allows for flexible control over the trade-off between content and style fidelity. By adjusting this parameter, the method can balance between maintaining the content of the original image while adapting its style to match the given style.
The authors evaluate CSTO through extensive experiments using various baseline methods and compare its performance to other state-of-the-art techniques. The results show that CSTO outperforms these baselines across different ranges of content and style fidelity, demonstrating its effectiveness in transferring styles while preserving the original content.
To better understand how CSTO works, the authors visualize the effects of adjusting γ on the image’s content and style. They find that as γ increases, the method transfers more of the style from the given image, while decreasing its content, and vice versa. This characteristic allows users to adjust the degree of style transfer according to their personal preferences.
The authors also investigate the impact of another parameter, τ, which controls the scaling of the attention map used in CSTO. They find that increasing τ enhances the performance of style transfer but with diminishing returns as τ grows larger. This modification effectively scales the attention temperature, enabling the method to focus more on the relevant parts of the image while ignoring less important details.
In summary, CSTO offers a novel approach to style transfer that balances content and style fidelity through flexible control over the trade-off parameter γ. The method’s effectiveness is demonstrated through extensive experiments, and its internal mechanics are visualized to help users understand how it works. By introducing τ scaling, CSTO further improves its performance, offering a more comprehensive solution for image style transfer.
Computer Science, Computer Vision and Pattern Recognition
Large-Scale Pre-Trained DMs Enable Efficient and Fidelity-Aware Style Transfer
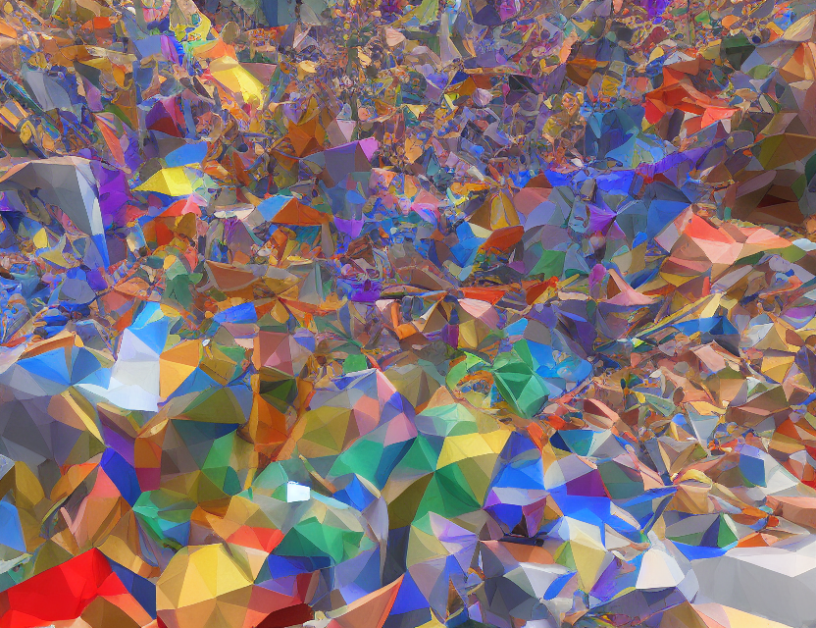