In this article, we explore the use of "grounding" in object detection tasks, which involves aligning the semantic meaning of phrases with visual regions in images. By combining both detection and grounding datasets, our proposed method, DINO, enhances the accuracy of object alignment compared to using only image-level alignment. We demonstrate the effectiveness of DINO by achieving better performance in novel classes and showing superiority over baseline models.
To understand how grounding works, imagine you’re trying to find a specific object in a cluttered room. Without any context, it would be challenging to locate the object, but with some hints, like the color or shape, it becomes much easier. Similarly, grounding provides contextual cues for object detection models to better identify objects in images.
Our approach involves resorting to a description-enriched concept dictionary to enhance the accuracy of object alignment. This dictionary contains general concepts extracted from multiple data sources and is used to align phrases with visual regions in images. By using this approach, we achieve better performance in novel classes and show that grounding offers limited help in fine-grained classes.
We also analyze the importance of bounding boxes in object detection tasks and find that accurate bounding box prediction is crucial for good performance. Unfortunately, some classes, like "Trailer," have limited performance due to inaccurate bounding box predictions, but we attribute this to label alignment with grounding DINO rather than box inaccuracy.
In summary, our proposed method, DINO, enhances the accuracy of object alignment by combining both detection and grounding datasets. By using a description-enriched concept dictionary, we achieve better performance in novel classes and show that grounding offers limited help in fine-grained classes. Grounding provides contextual cues for object detection models, making it easier to locate objects in images, and accurate bounding box prediction is crucial for good performance.
Computer Science, Computer Vision and Pattern Recognition
Enhancing Object Detection with Phrase Grounding: A Survey
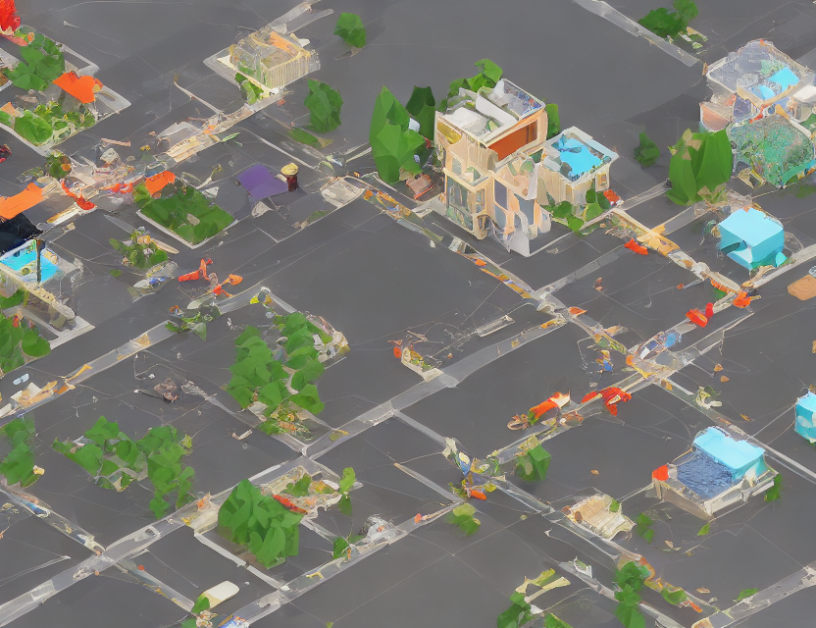