In this research, we conducted an ablation study to evaluate two main contributions in the field of text-to-image synthesis. Our goal was to improve the quality and efficiency of text-to-image generation models. We used a diffusion model, which is a type of neural network, to generate images from text descriptions.
Firstly, we studied the impact of a new method called Attention Normalization on our proposed model. This method allows the model to focus more on the relevant parts of the input text when generating images. As shown in Figure 9, Attention Normalization significantly improves the quality of generated images compared to the original model.
Secondly, we tested different acceleration methods for training and inference, including large-parameter fine-tuning and adapting-free methods. We found that these methods reduce computational costs and inference time, but they also come with a trade-off of higher training costs and potential limitations in achieving precise quality control.
Our findings demonstrate the effectiveness of Attention Normalization in improving the quality of text-to-image synthesis. Additionally, we showed that there are different approaches to accelerate the training and inference process, each with its advantages and disadvantages. By combining these techniques, we can create more efficient and effective text-to-image generation models.
In summary, our ablation study aimed to improve the quality and efficiency of text-to-image synthesis by exploring new methods and evaluating their impact on the performance of the model. Our findings show that Attention Normalization is a promising technique for improving the quality of generated images, while acceleration methods can reduce computational costs and inference time but come with trade-offs. These insights can help researchers and practitioners develop more advanced text-to-image generation models in the future.
Computer Science, Computer Vision and Pattern Recognition
Accelerating Diffusion Models with Progressive Distillation and Attention Normalization
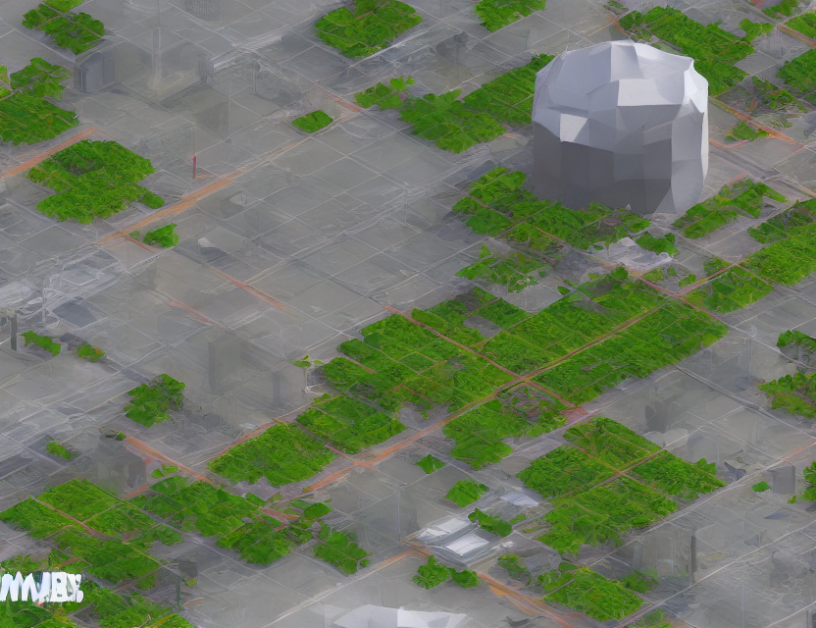