In this article, the authors explore the challenges of developing models that can accurately understand and reason about visual content, specifically in the context of image captioning tasks. They highlight how traditional semantic segmentation approaches are insufficient for handling complex queries with intricate expressions or longer sentences, as they fail to account for the broader context and relationships within a scene. To address this issue, the authors propose TIGEr (Text-to-image Grounding for Image Caption Evaluation), a novel approach that integrates text-to-image grounding with image caption evaluation. The proposed method leverages advances in natural language processing to better understand and interpret complex queries, enabling more accurate image captioning outputs.
The authors begin by discussing the limitations of traditional semantic segmentation approaches, which are unable to comprehend and reason about the broader context within a scene. They argue that this oversight leads to inaccurate image captions, as models fail to consider the complex relationships and expressions present in the visual content. To address this issue, the authors propose TIGEr, an innovative approach that combines text-to-image grounding with image caption evaluation.
The proposed method utilizes advances in natural language processing to better understand and interpret complex queries. By leveraging a chain-of-thought approach, TIGEr can identify the relationships between different elements within a scene and generate more accurate image captions. The authors demonstrate the effectiveness of their approach through experiments on several benchmark datasets, showing improved performance compared to existing methods.
In conclusion, this article highlights the challenges of developing models that can accurately understand and reason about visual content, specifically in the context of image captioning tasks. The proposed TIGEr method offers a novel solution by integrating text-to-image grounding with image caption evaluation, enabling more accurate and comprehensive image captions. By leveraging advances in natural language processing, this approach can better understand complex queries and generate more accurate outputs, paving the way for more sophisticated visual reasoning models in the future.
Computer Science, Computer Vision and Pattern Recognition
Augmenting False-Premise Referring Expressions with Generative Language Models
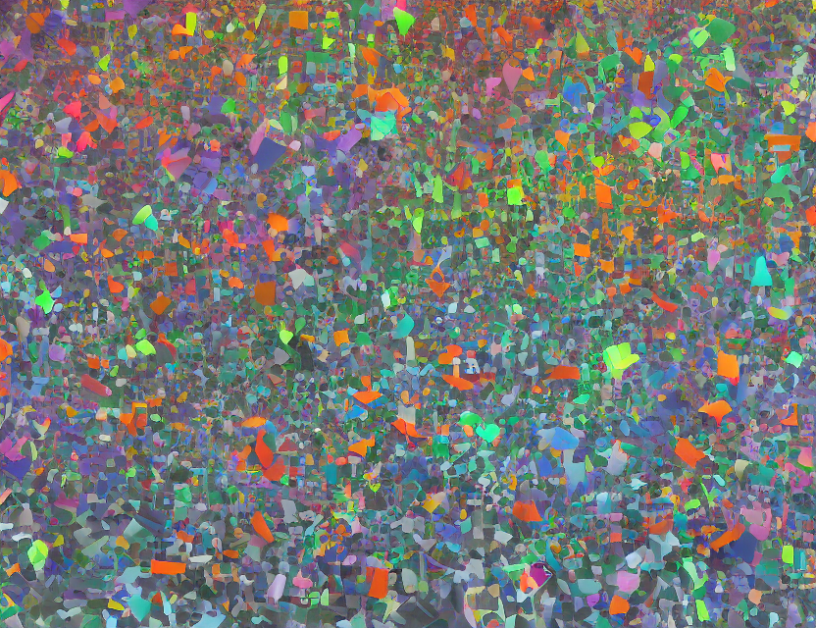