In this article, the authors propose using language models (LLMs) to enhance the visual representation of archaeological artifacts. They aim to alleviate two main issues in the field: noisiness and knowledge deficiency. LLMs can act as both an information extractor and an external knowledge base, retrieving vital attributes and completing missing ones based on archaeological expertise.
The authors explain that while some artifact attributes like "name," "time period," and "size" are often available in museum resources, the specific "material," "shape," and "pattern" need to be extracted or derived from the raw description of the object. Additionally, the classified "type" of an artifact determines certain fundamental aspects of its appearance, which can be defined by the generic definition of this artifact-type (i.e., "type definition").
To tackle these challenges, the authors suggest using text-to-image synthesis, which has shown potential in recreating visual images of ancient artifacts. They highlight that while diffusion models have demonstrated significant capabilities in generating photorealistic images based on a given text prompt in open-domain problems, they struggle to produce promising results in specialized archaeological studies due to limited data and domain knowledge.
To overcome these limitations, the authors propose utilizing LLMs as a powerful tool for visualizing ancient artifacts. They explain that LLMs can learn the implicit knowledge in textual information and generate images that match the shape, patterns, and details of the artifacts. By combining LLMs with diffusion models, the authors aim to create an efficient and reliable method for generating accurate visual representations of archaeological artifacts.
In conclusion, this article presents a novel approach to enhancing the visual representation of archaeological artifacts by leveraging the power of language models. The proposed method has the potential to revolutionize the field of archaeology by providing historians with new visual angles to study the past and enabling people to connect with their cultural heritage.
Computer Science, Computer Vision and Pattern Recognition
Deep Learning for Image Recognition: A Survey of Recent Approaches
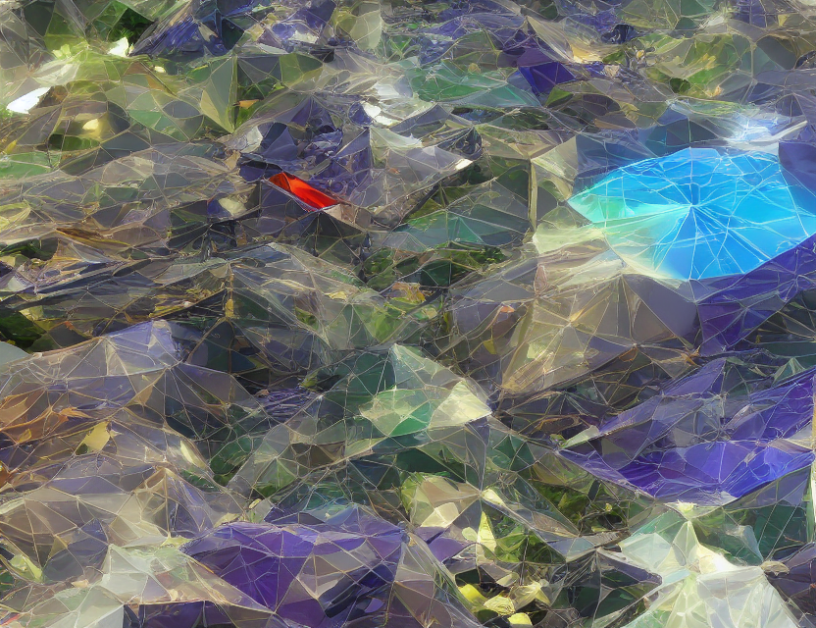