In this article, the authors propose a novel approach to image editing that leverages the power of Generative Adversarial Networks (GANs). They aim to create a more efficient and controllable method by transforming the latent space of the GAN into a compact and semantic space. This allows artists to manipulate individual attributes of an image without affecting others, making editing simpler and more intuitive.
The authors begin by acknowledging that most GANs are over-parametrised, meaning they have too many dimensions that influence the generated images. To address this issue, they apply a technique called Principal Component Analysis (PCA) to extract the most important attributes of the latent space. These attributes are then transformed into a compact and semantic space using a sub-space extraction method.
The authors evaluate their approach using three quantitative metrics: identity conservation during an edit, attribute variation during an edit, and well-edited image rate. They find that their method outperforms state-of-the-art methods in these areas while also providing a more efficient editing process.
To understand the compact and semantic latent space, imagine a digital artist working on a painting. The artist wants to change the color of a specific object without affecting others in the image. By transforming the latent space into this new space, the artist can now manipulate individual attributes independently, making the editing process more straightforward and intuitive.
In conclusion, the authors propose a novel approach to image editing that leverages the power of GANs while demystifying the complex concepts involved. Their compact and semantic latent space makes editing simpler and more intuitive, allowing artists to focus on their creativity rather than navigating complex algorithms.
Computer Science, Computer Vision and Pattern Recognition
Disentangled Image Editing via Compact and Semantic Latent Space
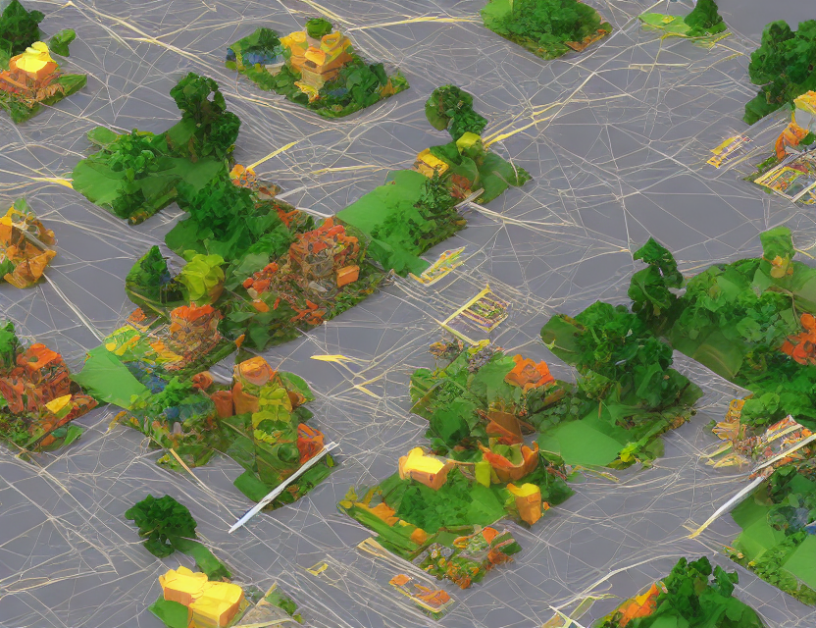