In this article, we explore a novel approach to extracting fact descriptions and making final predictions in Indian Law Journal Predictor (ILJP). The proposed framework enhances the semantics from both aggregated chunk and document-level semantics by creating a concise view of the fact description as per the original court case document structure. This concise extraction module is crucial in avoiding data loss due to extracting semantics at multiple levels.
The article explains how the Concise Extraction (CE) module works by appending the document summary at the last few lines and applying attention to concentrate on the main words impacting subsequent prediction. The final outcome prediction is made through sigmoid using the resultant enriched semantics containing essential key phrases impacting decision-making.
The article also discusses the procedure for implementing the proposed framework, which involves chunking the fact description into smaller chunks, processing each chunk to get word embeddings, and then checking the semantics extraction. The approach helps in understanding through transformer ex: XLNET and draws chunk-level semantics by applying the divide-and-conquer approach and extracting document-level semantics dynamically.
The article concludes by highlighting the significance of the proposed framework in making analysis of complex unstructured case documents more accessible and easier to comprehend. The concise summary of the article focuses on demystifying complex concepts using everyday language and engaging metaphors or analogies to capture the essence of the article without oversimplifying.
Computation and Language, Computer Science
Enhancing Predictive Accuracy of Semantics-Based Decision Making through Novel Concise Extraction
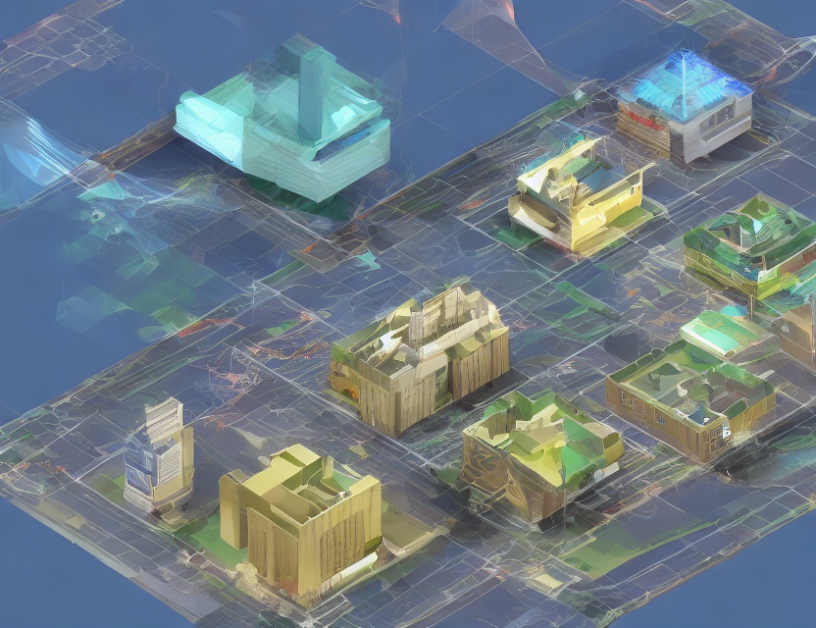