Fairness in machine learning is a critical concern in today’s society, as these algorithms are increasingly being used to make decisions that affect people’s lives. The article provides a comprehensive survey of the different approaches to fairness in machine learning, including individual and group fairness, counterfactual fairness, and human-centered approaches.
Individual fairness focuses on ensuring that similar individuals are treated similarly, regardless of their group membership. Group fairness, on the other hand, considers the overall distribution of outcomes across different groups. Counterfactual fairness involves assessing the fairness of a model by comparing the outcomes it produces to what they would be if the model were fair.
Human-centered approaches prioritize human involvement in the fairness evaluation process, recognizing that humans are essential for ensuring fairness in machine learning systems. The article also discusses challenges and open research directions in this field, including addressing the gap between individual and group fairness, developing practical methods for counterfactual fairness, and improving human-centered approaches to fairness evaluation.
To ensure fairness in machine learning, the article highlights the importance of considering the ethical and social implications of these systems, as well as involving diverse stakeholders in the development and evaluation process. By adopting a human-centered approach to fairness, we can develop more responsible and equitable AI systems that benefit everyone in society.
Artificial Intelligence, Computer Science
Fairness in Machine Learning: A Survey of Human-in-the-Loop Approaches
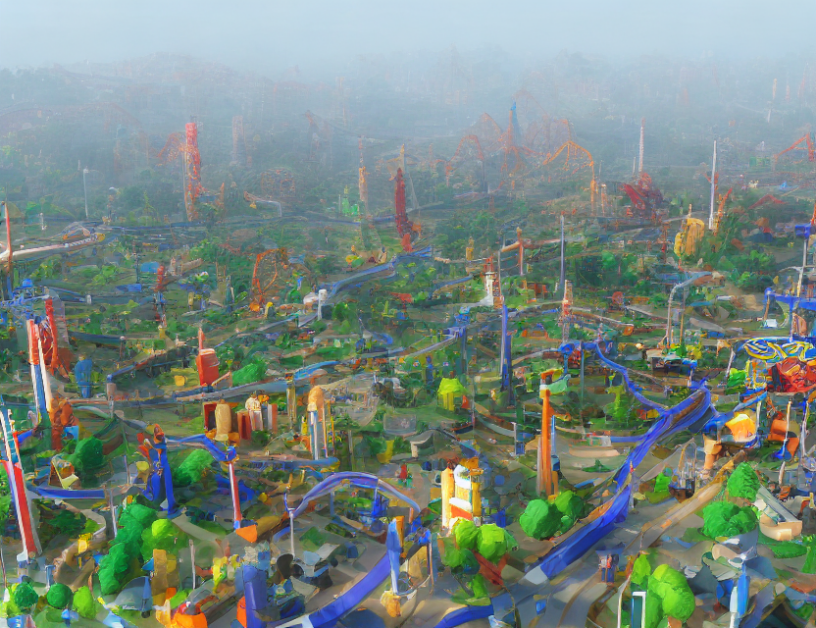