In this article, the authors propose a new approach to few-shot learning called "adaptive loss functions." They argue that traditional methods rely on simplistic criteria such as selecting maximal values from source images or using a single loss function for all tasks, which can lead to a lack of adaptability and oversimplify the problem.
To address this issue, they introduce an "adaptive fusion loss proposal module" that learns to propose different loss functions tailored to specific scenarios and tasks. This module is designed to be lightweight and efficient, allowing it to be easily integrated into existing few-shot learning frameworks.
The authors also propose a streamlined fusion module and a two-branch network dedicated to reconstruction, which work together to improve the overall performance of the framework. The learning process is divided into three stages, with each stage using a different loss function to optimize the fusion and reconstruction modules.
In summary, the proposed adaptive loss functions offer a more flexible and effective approach to few-shot learning by adapting to different scenarios and tasks. By proposing tailored loss functions and streamlining the fusion process, this framework improves upon traditional methods and demonstrates better performance in various applications.
Computer Science, Computer Vision and Pattern Recognition
Fine-Tuning Loss Proposal for Improved Fusion: A Novel Approach
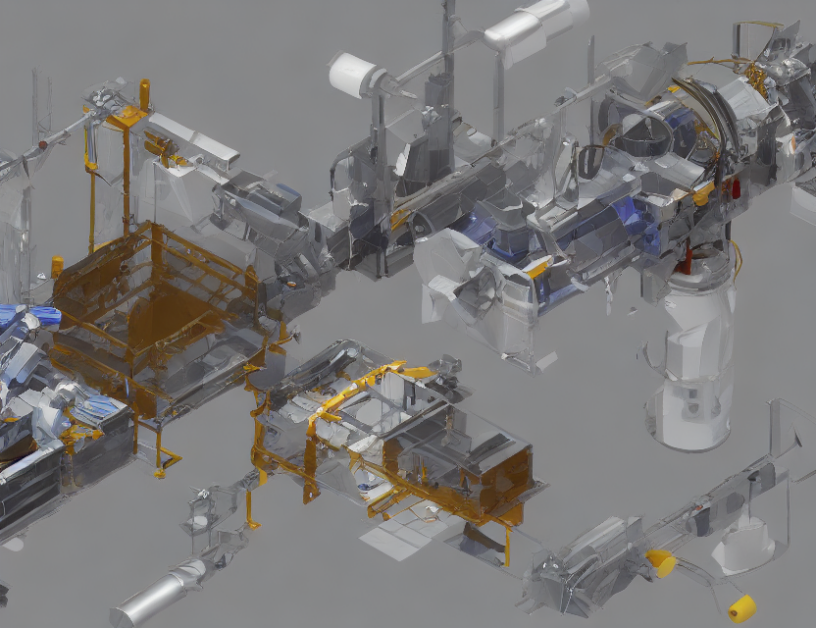