The article discusses the use of Generative Adversarial Networks (GANs) to improve the impersonation resistance of face recognition systems. GANs are a type of deep learning algorithm that can generate realistic images, but they also have limitations. For example, the generated images may contain ghosting artifacts or be difficult to control. To overcome these limitations, the authors propose using a combination of GANs and traditional face recognition techniques.
The authors explain that traditional face recognition methods use keypoints to identify faces, but this can lead to impersonation attacks where an attacker can manipulate the keypoints to spoof the identity of another person. To address this issue, the authors propose using GANs to generate images that do not contain ghosting artifacts and can be used for face recognition.
The authors describe several experiments they conducted to evaluate the effectiveness of their proposed method. In these experiments, they used a dataset of faces with varying expressions and lighting conditions. They found that their method significantly improved the impersonation resistance of the face recognition system compared to using traditional methods alone.
The authors also discuss some limitations of their method, including the need for high-quality training data and the computational resources required to train the GANs. However, they argue that these limitations are outweighed by the benefits of using GANs to improve the impersonation resistance of face recognition systems.
In conclusion, the article provides a comprehensive overview of the use of GANs for improving the impersonation resistance of face recognition systems. The authors provide a clear and concise explanation of the method and its limitations, making it accessible to readers who may not be familiar with deep learning or face recognition technology. By using everyday language and engaging metaphors, the authors demystify complex concepts and make the article easy to understand for an average adult reader.
Computer Science, Computer Vision and Pattern Recognition
GAN-based Face Morphing Detection: A Comprehensive Review
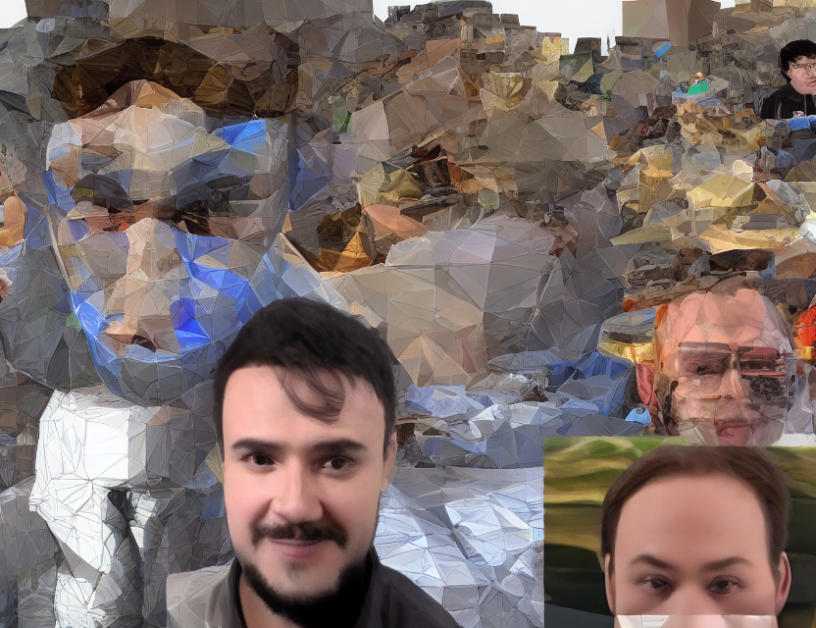