In this article, the authors propose a novel approach to improve the understanding of 3D scenes by introducing a relation-aware token, which enables the model to perceive the spatial relationships among objects in a scene. The token is derived from two types of data: scene-aware object captioning and scene-level question-answering. These data sources provide the model with information about the objects in the scene and their positions relative to each other.
To train the model, the authors construct single-turn QA pairs where the question asks for a brief description of an object in a 3D scene. After alignment, they fine-tune the model on various downstream tasks, allowing it to adapt to different formats and ultimately achieve improved performance. This fine-tuning process enhances the model’s overall perception and reasoning abilities within the 3D scene.
The authors compare their approach with previous methods that rely solely on object identifiers in the response. They demonstrate that their relation-aware token enables the model to freely reference objects when describing a complex 3D scene, leading to better performance in downstream tasks such as scene understanding and object recognition.
The key insight behind this approach is that by using a relation-aware token, the model can grasp the spatial relationships among objects in a scene more effectively. This allows it to better understand the context of each object and its position relative to other objects in the scene. As a result, the model can provide more accurate and informative responses when describing complex 3D scenes.
In summary, the authors propose a novel approach to improve 3D scene understanding by introducing a relation-aware token that enables the model to perceive spatial relationships among objects in a scene. This approach is demonstrated to be effective through experiments that compare it with previous methods. By providing a more accurate and informative response, this approach can help machines better understand complex 3D scenes and improve their overall performance in downstream tasks.
Computer Science, Computer Vision and Pattern Recognition
Improved Scene Captioning with End-to-End Fine-tuning and Modular Knowledge Transfer: A Comprehensive Study
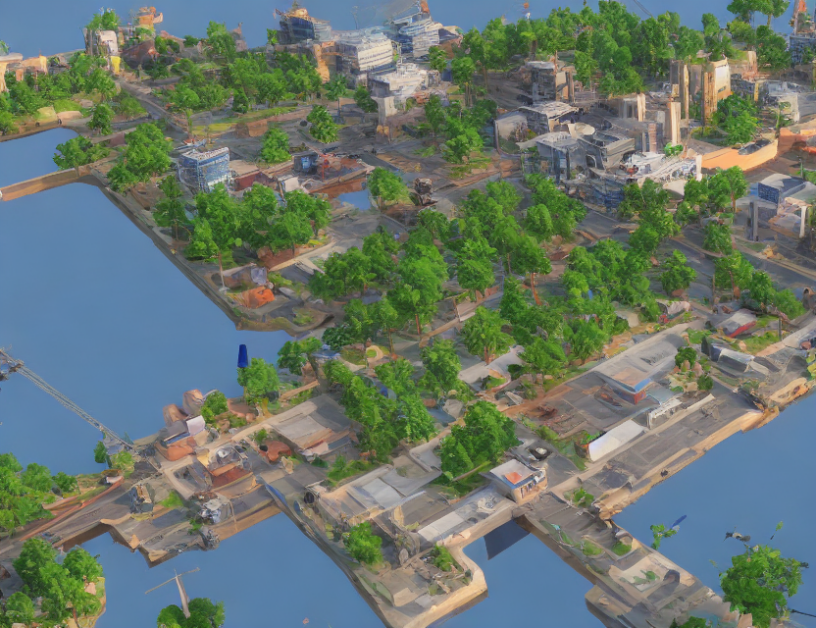