In this article, the authors propose a novel approach to multimodal sentiment analysis that leverages the strengths of both natural language processing (NLP) and computer vision techniques. The proposed method, called DQPSA, employs a two-stage pre-training framework that combines the robustness of NLP with the visual information of images. This approach allows for better performance in semantic information and sentiment analysis, resulting in improved accuracy compared to traditional methods that focus solely on token-level visual information.
The authors explain that existing methods often struggle to effectively analyze multimodal data due to the complex relationships between natural language and visual features. To address this challenge, DQPSA introduces a novel attention mechanism that selectively focuses on relevant visual regions based on the sentiment of the input text. This allows the model to effectively capture both the semantic meaning and sentiment of the input data, leading to improved performance in sentiment analysis tasks.
The authors evaluate their proposed method on two political Twitter datasets, demonstrating competitive results compared to state-of-the-art methods. They also provide a detailed analysis of the attention mechanisms used by DQPSA, showing how they improve the model’s ability to capture sentiment information in multimodal data.
Overall, the authors’ proposed approach represents a significant step forward in multimodal sentiment analysis, demonstrating the potential benefits of combining NLP and computer vision techniques. Their novel attention mechanism and two-stage pre-training framework provide a robust foundation for improving the accuracy of multimodal sentiment analysis models.
Artificial Intelligence, Computer Science
Joint Modeling of Input Sequences and Target Labels for Aspect-Sentiment Analysis
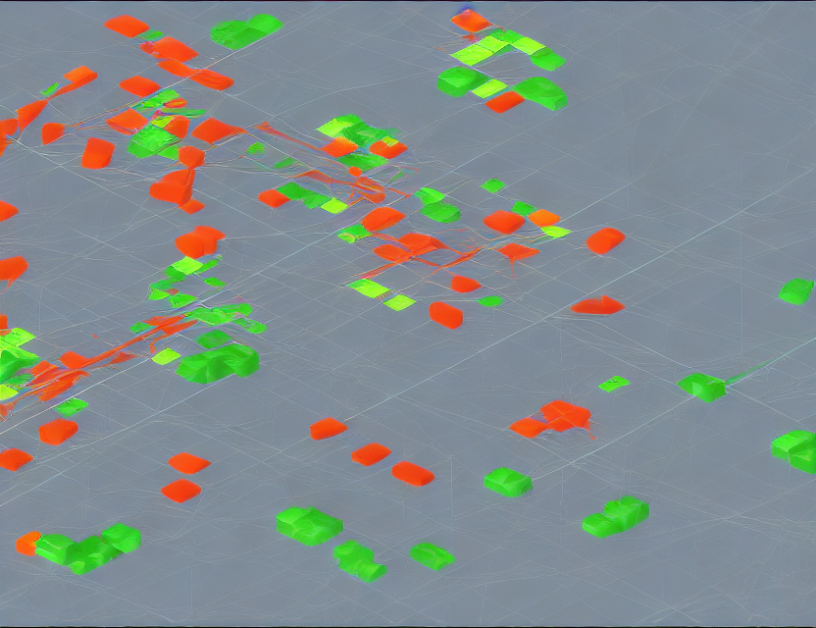