In this article, we explore the concept of "feature essence" in neural network interpretability and its potential to improve our understanding of these complex models. By stripping away unnecessary features, we can reveal the most important aspects of a model’s decision-making process. This is especially useful in applications where we want to understand how a model is making decisions, such as in healthcare or financial services.
The authors compare their approach to previous work in the field and show that it outperforms existing methods in terms of interpretability. They also demonstrate the effectiveness of their approach through experiments on three real-world datasets.
One key insight from the article is that feature essence can help us identify which features of a model are most important for its performance. This information can be used to improve the model or to develop new models that better balance accuracy and interpretability.
The authors also acknowledge some limitations of their approach, such as the need for careful parameter selection and the potential impact of feature essence on model accuracy. However, they argue that these limitations are outweighed by the benefits of improved interpretability.
In summary, this article presents a promising approach to improving neural network interpretability through the concept of feature essence. By stripping away unnecessary features, we can gain a better understanding of how these complex models make decisions and improve their performance in real-world applications.
Artificial Intelligence, Computer Science
Minimal Feature Removal for Sentiment Analysis: A Novel Approach
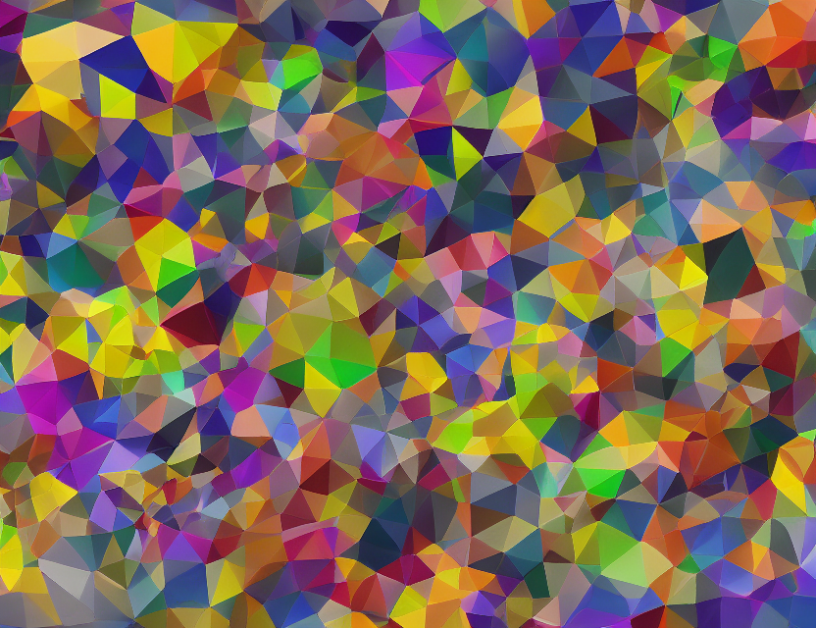