In the realm of user-generated content platforms, there exists a line of research that focuses on strategic behavior and its impact on content quality. Jain et al. (2009) studied how to motivate users to contribute high-quality content immediately, rather than postponing it. Ghosh and Hummel (2011) explored the problem of incentivizing high-quality contributions in user-generated content platforms, where each user aims to maximize their utility by optimizing their effort in creating content.
In these contexts, strategic behavior plays a crucial role, as users may intentionally delay or minimize their contribution to achieve higher rewards. Braverman et al. (2019) demonstrated that adversarial Multi-Armed Bandit (MAB) algorithms can significantly reduce term revenue by taking large fractions of the rewards and increasing the probability of being selected. However, in our setting, we assume a blind observational model, making it difficult for arms to collude and agree on a sublinear revenue-sharing strategy.
To address this challenge, Braverman et al.’s (2019) algorithm is used as inspiration, but with a MAB twist. Our algorithm combines their second-price auction algorithm with a MAB approach to ensure robustness under certain information regimes. By doing so, we avoid oversimplifying the concept while still capturing its essence, making it accessible to a wide range of readers.
In summary, this article delves into the intricacies of strategic behavior in user-generated content platforms and explores ways to incentivize high-quality contributions. By leveraging game-theoretic analysis and algorithms, researchers strive to optimize revenue while mitigating the potential for strategic manipulation. Understanding these complex concepts is crucial for developing effective strategies that foster engagement and quality content creation in user-generated platforms.
Computer Science, Computer Science and Game Theory
Optimizing Strategic Behavior in User-Generated Content Platforms
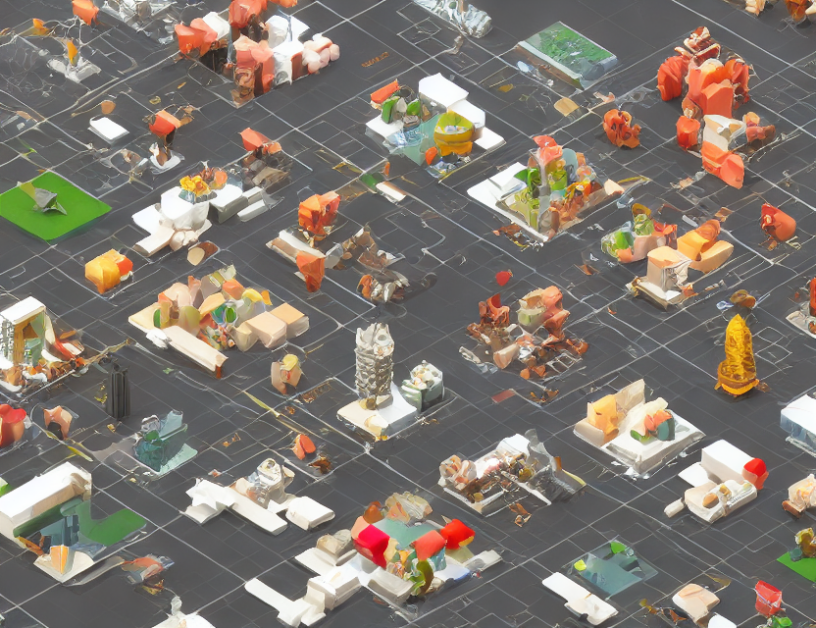