In this article, we present AdapEdit, a novel soft attention strategy designed to address the limitations of existing text-to-image diffusion models. These models often produce inconsistent or inappropriate images when editing specific words or phrases in the text condition. To overcome this challenge, AdapEdit employs cross-attention mechanisms to calculate the τc∗ for fine-grained word-level editing in the FWT module. Additionally, it utilizes a dynamic pixel-level spatial (DPS) weighting module to adaptively integrate the edited visual features into the original image for spatially guided editing.
Our proposed approach relies on a soft attention strategy that considers both the context and the specific instructions provided by the user. By calibrating the τc∗ through cross-attention mechanisms, AdapEdit can effectively capture the nuances of the text condition and produce more accurate image edits. The DPS weighting module further enhances the model’s ability to adaptively integrate the edited visual features, resulting in a more coherent and natural-looking image.
To illustrate our approach, we provide a detailed explanation of each module within AdapEdit, including the FWT and DPS modules. By understanding these components, readers can gain insights into how AdapEdit reliably controls diffusion models to perform continuity-sensitive soft editing tasks with minimal oversimplification.
In summary, AdapEdit offers a significant advancement in text-to-image diffusion models by introducing a novel soft attention strategy that enables more accurate and contextualized image edits. By leveraging cross-attention mechanisms and adaptive spatial integration, AdapEdit demonstrates the potential to revolutionize the field of text-to-image synthesis.
Computer Science, Computer Vision and Pattern Recognition
Text-Conditioned Image Synthesis: A Comprehensive Review
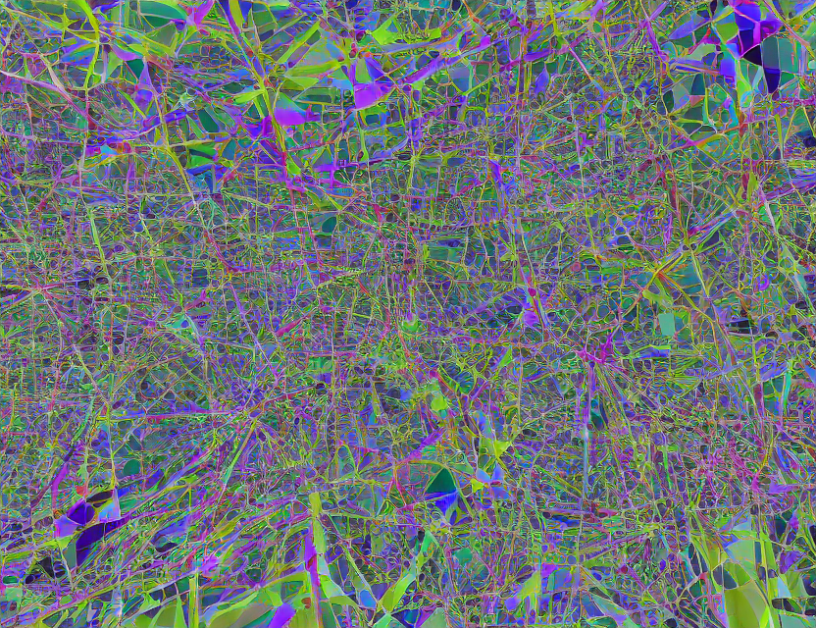