In this article, the authors propose a new method for generating high-fidelity 3D scenes from text inputs. They build upon existing text-to-3D object approaches, such as score distillation sampling, and extend them to create global 3D representations that can capture scenes with objects scattered throughout. To achieve this, they introduce a hybrid representation that marries the locality of objects with the globality of scenes.
The authors explain that generating 3D scenes from text is challenging because scenes are inherently global while objects are local. They propose to address this challenge by introducing a hybrid representation that combines the strengths of both local and global representations. This hybrid representation allows for the optimization of scene-aware 3D representations, which can be used to generate high-fidelity 3D scenes from text inputs.
The authors evaluate their proposed method using several metrics and show that it achieves state-of-the-art performance in text-to-3D scene generation. They also demonstrate the effectiveness of their approach by synthesizing 3D scenes based on a wide range of user-provided text prompts, while accommodating specific user preferences for 3D assets and seamlessly arranging the selected objects within the scenes.
To configure the layout of the scene, the authors use Particle Swarm Optimization, which is presented in detail in the article. They also incorporate a pre-trained RGBD panorama diffusion model to provide additional guidance on occlusion mitigation.
In summary, SceneWiz3D is a novel approach that enables the generation of high-fidelity 3D scenes from text inputs by combining the strengths of local and global representations. The proposed method achieves state-of-the-art performance in text-to-3D scene generation and demonstrates its effectiveness through several evaluation metrics.
Computer Science, Computer Vision and Pattern Recognition
Text-to-3D: A Comprehensive Review of Methods and Techniques
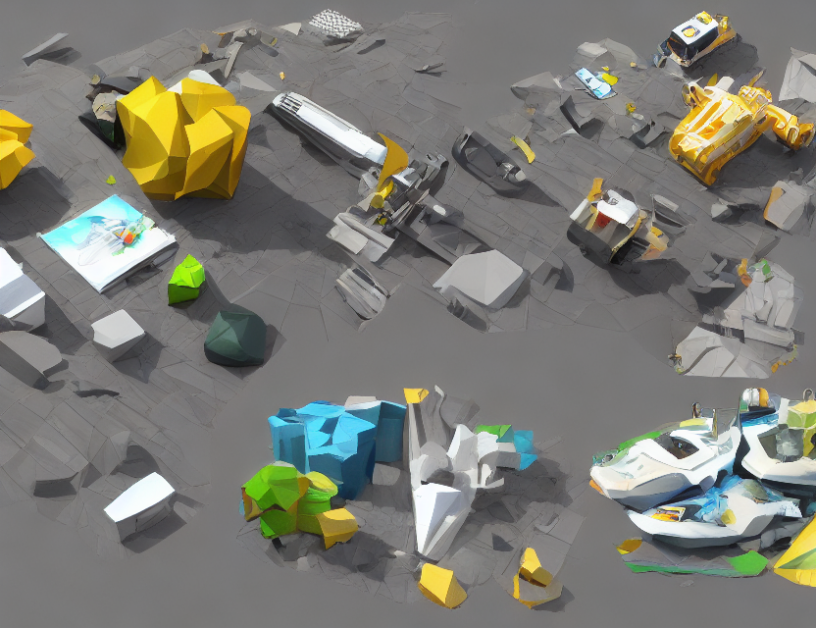