In this article, the authors propose a novel approach to text image super-resolution using a diffusion model that integrates text prior information. The proposed method, called Text Diffusion Model (TDM), aims to enhance the quality and accuracy of text images by leveraging the power of diffusion models.
Diffusion models have been widely used in image processing tasks due to their ability to enrich and enhance image details. However, most existing methods neglect the importance of text information, leading to suboptimal performance in text image super-resolution. To address this limitation, TDM integrates text prior information into the diffusion model, allowing it to capture the underlying text structure and semantics.
The proposed method consists of two main components: an Image Diffusion Model (IDM) and a text recognition model. IDM is responsible for image super-resolution through a diffusion reverse process conditioned on both low-resolution (LR) text images and estimated text prior. The text recognition model, on the other hand, estimates the text sequence from LR text images as a prior in every diffusion step.
To guarantee the accuracy of restored text images, TDM incorporates three key aspects to constrain text image super-resolution: text aware loss, text recognition prior, and text structure prior. These constraints ensure that the restored text images are not only visually pleasing but also semantically accurate.
The authors conduct an ablation study to validate the effectiveness of TDM by comparing it with existing methods. The results demonstrate that TDM outperforms its counterparts in terms of both image quality and accuracy, proving its potential in enhancing text image super-resolution.
In summary, TDM is a novel approach to text image super-resolution that leverages diffusion models to enhance both image quality and accuracy. By integrating text prior information into the diffusion model, TDM can capture the underlying text structure and semantics, leading to more accurate and visually pleasing restored text images.
Computer Science, Computer Vision and Pattern Recognition
Text-to-Image Synthesis with Diffusion Models: A Comprehensive Review
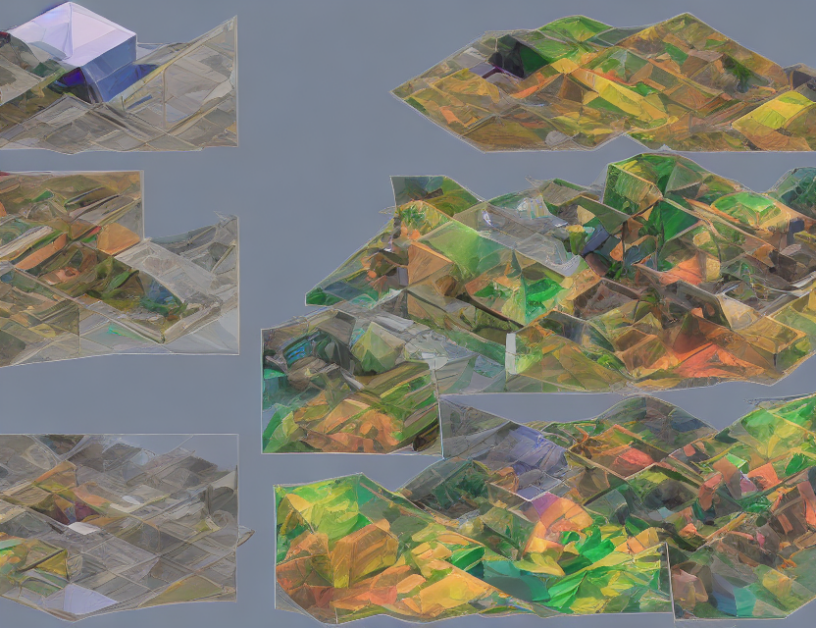