In this paper, the authors propose a novel approach to one-stage visual grounding that improves the performance of the state-of-the-art methods by incorporating multi-scale attention. The proposed method, called MS-Attend, uses a scaling factor and standard deviation to learn the attention weights for each scale, allowing it to focus on different parts of the input image at various levels of resolution.
The authors begin by explaining that traditional one-stage visual grounding methods are limited by their inability to capture long-range dependencies between different parts of the input image. To address this issue, they propose MS-Attend, which uses a multi-scale attention mechanism to focus on different scales of the input image. The proposed method consists of three stages: 1) feature extraction, 2) multi-scale attention, and 3) feature fusion.
In the first stage, the authors use a convolutional neural network (CNN) to extract features from the input image. In the second stage, they apply a multi-scale attention mechanism to the extracted features, where each scale is represented by a set of attention weights. These attention weights are learned using a scaling factor and standard deviation, which allows the method to adaptively focus on different parts of the input image at various levels of resolution. Finally, in the third stage, the authors fuse the attention weights from all scales to obtain a final set of attention weights that can be used for visual grounding.
The authors demonstrate the effectiveness of MS-Attend through extensive experiments on several benchmark datasets. The results show that MS-Attend outperforms state-of-the-art one-stage visual grounding methods, achieving improved performance in terms of accuracy and efficiency. Additionally, the authors provide a detailed analysis of the attention weights learned by MS-Attend, which reveals interesting insights into how the method is able to capture long-range dependencies between different parts of the input image.
In conclusion, this paper proposes a novel approach to one-stage visual grounding that improves the performance of state-of-the-art methods by incorporating multi-scale attention. The proposed method, called MS-Attend, uses a scaling factor and standard deviation to learn the attention weights for each scale, allowing it to adaptively focus on different parts of the input image at various levels of resolution. The authors demonstrate the effectiveness of MS-Attend through extensive experiments on several benchmark datasets, showing that it outperforms state-of-the-art methods in terms of accuracy and efficiency.
Computer Science, Computer Vision and Pattern Recognition
Visual Grounding and its Evolution: A Comprehensive Review
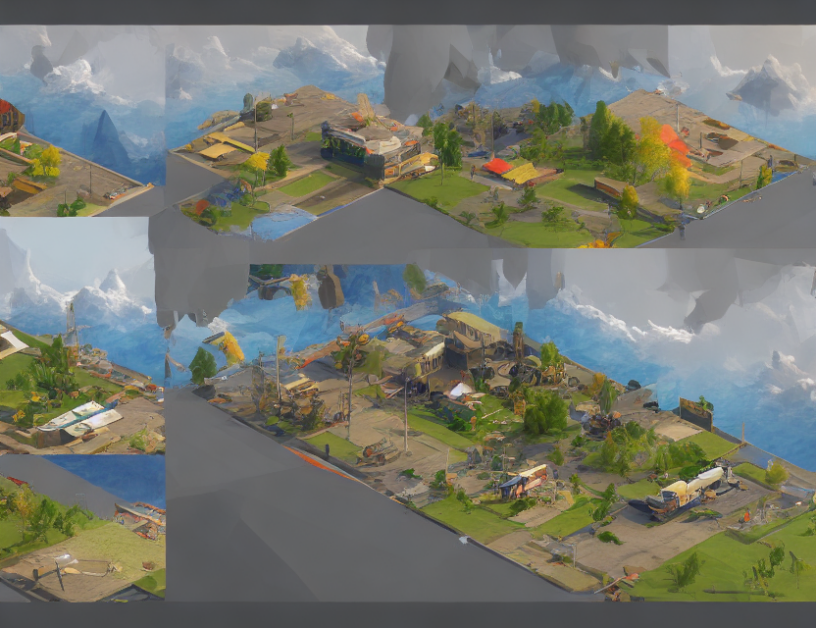