In this article, we present a comprehensive database of video sequences with various artifacts and natural scenes to evaluate the quality of video content. Our approach is designed to provide explainability by using a language-prompted method that helps assessors understand the factors affecting video quality. The database contains 60 source sequences, each with a unique artifact or natural scene, and 120 heavily compressed versions of these sources. We also include 10 pristine sequences for comparison.
To create this database, we selected a variety of artifacts such as motion blur and dark scenes, which can degrade video quality when present in excess. For each artifact, we collected 10 source videos with the corresponding heavily compressed versions to study their impact on perceived quality. Our goal is to develop an objective assessment method that can accurately evaluate the quality of video content under different conditions.
Imagine you’re watching a movie, and suddenly, the characters start moving in a blurry manner. This can be frustrating, especially if it happens frequently throughout the film. Motion blur is one of the artifacts we included in our database, as it can affect video quality significantly. Another common issue is dark scenes, where an area of the image appears underexposed, making it difficult to see details.
To address these challenges, we developed a language-prompted approach that enables assessors to understand the factors contributing to poor video quality. By using natural language processing techniques, we can identify the specific artifacts or scenes causing degradation and provide explanations for each one. This transparency helps developers improve their video content quality more efficiently.
In conclusion, our database provides a comprehensive collection of artifact-affected and natural sequences to evaluate video quality in a more explainable manner. Our language-prompted approach enables assessors to understand the factors contributing to poor video quality, which can lead to better content optimization for improved viewing experiences.
Computer Science, Computer Vision and Pattern Recognition
Assessing Video Quality: A Comprehensive Review
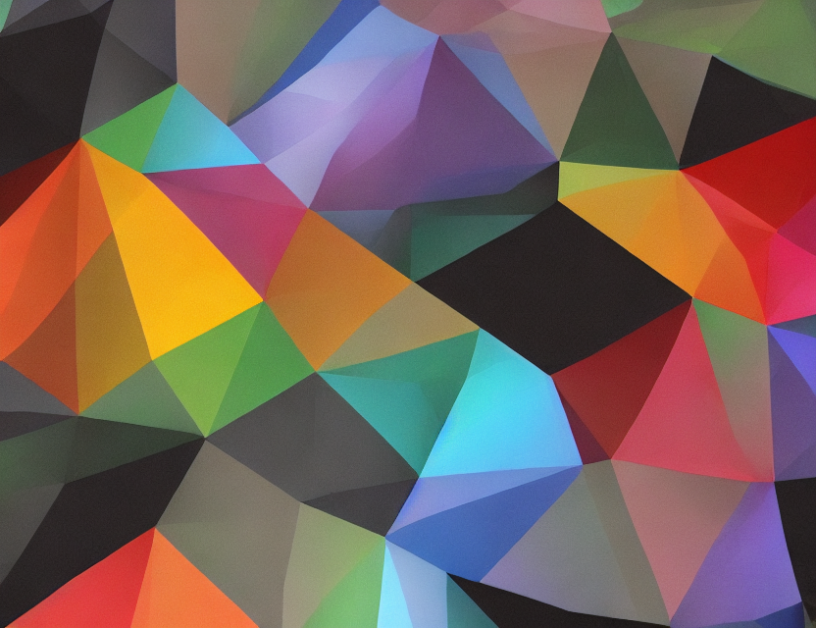