In this article, the authors explore the challenges of style transfer in visual narratives, such as comics and manga. These mediums have distinct art styles that vary across several aspects, making it crucial to preserve narrative semantics during style transfer. The authors introduce the notion of comprehension-preserving style transfer (CPST), which requires both traditional metrics of style transfer and metrics of narrative comprehension.
To evaluate CPST, the authors use visual story-cloze tests, similar to those used in computational cognition of narrative systems. These tests assess whether the style transfer process preserves coherence between panels and maintains narrative semantics. The authors also present an annotated dataset of comics and manga, along with initial algorithms that utilize separate style transfer modules for visual, textual, and layout parameters.
To understand the connection between style and narrative semantics, consider a recipe book with pictures of dishes. The visual style of each dish conveys its taste, texture, and aroma, while the accompanying text provides information on ingredients, cooking time, and nutritional value. Just as a chef must balance flavors and presentation to create a delicious meal, style transfer in visual narratives must reconcile artistic choices with narrative communication.
The authors’ approach to CPST involves analyzing the relationships between entities shared between panels, as well as panel layouts, to ensure that stylistic changes do not disrupt narrative coherence. By combining traditional style transfer metrics with narrative comprehension evaluations, CPST provides a more comprehensive understanding of style transfer in multi-modal visual narratives.
In summary, this article advances the field of style transfer by addressing its limitations in multi-modal visual narratives, such as comics and manga. By prioritizing narrative semantics alongside traditional style transfer metrics, CPST creates a more comprehensive understanding of how artistic choices impact storytelling.
Computer Science, Computer Vision and Pattern Recognition
Comprehensive Style Transfer for Multi-Modal Narratives
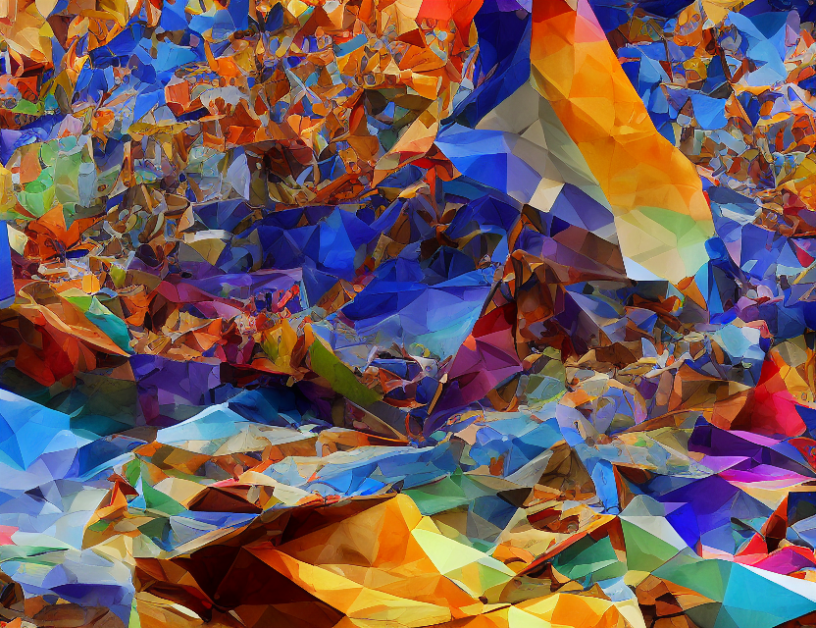