Counting livestock can be a daunting task, especially when done manually. In the livestock industry, it’s crucial to have an accurate count of the animals to determine their value and health. However, manual counting can lead to errors, as each cow is priced at around $1000. This article discusses the challenges of counting livestock and how machine learning algorithms can help improve accuracy.
The article highlights several experiments conducted to evaluate the performance of different machine learning models in counting livestock. The results show that while some models perform well in detecting and segmenting cows, there is still room for improvement in tracking and estimating their numbers accurately. The authors note that this gap in performance can be attributed to various factors, including the complexity of the task, the variability of cow positions, and the difficulty in distinguishing between cows and dirt background.
To address these challenges, the article suggests developing more advanced methods to improve detection, segmentation, and tracking of cows. The authors argue that this will motivate the community to develop and evaluate various types of machine learning models to tackle these challenges.
In conclusion, accurate counting of livestock is essential in the industry, and machine learning algorithms can help improve accuracy. However, there are still challenges that need to be addressed to achieve better performance. Developing more advanced methods can help overcome these challenges and ensure a more accurate count of livestock.
Computer Science, Computer Vision and Pattern Recognition
Dataset and Evaluation Metrics for Animal Behavior Understanding
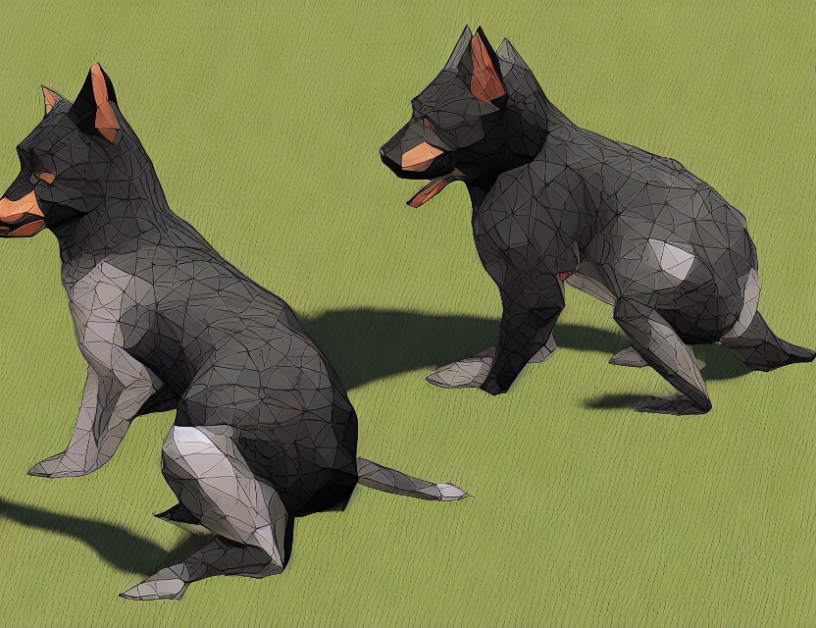