In this article, we delve into the realm of multimodal learning, specifically focused on human motion generation using textual prompts as a means to create diverse and coherent motions. The authors present a detailed analysis of their proposed approach, which combines CLIP [48] with a tailored flow matching model to generate high-quality motions that align with the given textual description.
To better understand this concept, imagine a magical wand that can transform text into motion. Just like how we use words to communicate complex ideas, our proposed approach leverages CLIP to encode text into a numerical representation that can be decoded into motion. The key difference is that instead of relying on a single modality (text), we incorporate both visual and linguistic information to create a multimodal understanding of the prompt.
The authors introduce the concept of "sampling trajectory rewriting," which allows them to manipulate the generated motions by sampling from a large dataset of human motions. This technique enables them to generate diverse and coherent motions that align with the given textual description. They also demonstrate the effectiveness of their approach by showcasing various failure cases, providing insights into the challenges of interpreting multiple fine-grained textual descriptions into motion.
To evaluate the quality of their generated motions, the authors employ a multimodal distance metric that measures the average Euclidean distance between the motion feature of each generated motion and the text feature of its corresponding description in the test set. A lower value indicates better multimodal distance, highlighting the effectiveness of their proposed approach.
Overall, this article provides a comprehensive overview of the authors’ proposed approach for human motion generation using textual prompts, offering valuable insights into the challenges and opportunities of multimodal learning in this domain. By leveraging both visual and linguistic information, their proposed approach has the potential to create more diverse and coherent motions that align with the given textual description.
Computer Science, Computer Vision and Pattern Recognition
Enhancing Human Motion Synthesis via Training-Free Editing Operations
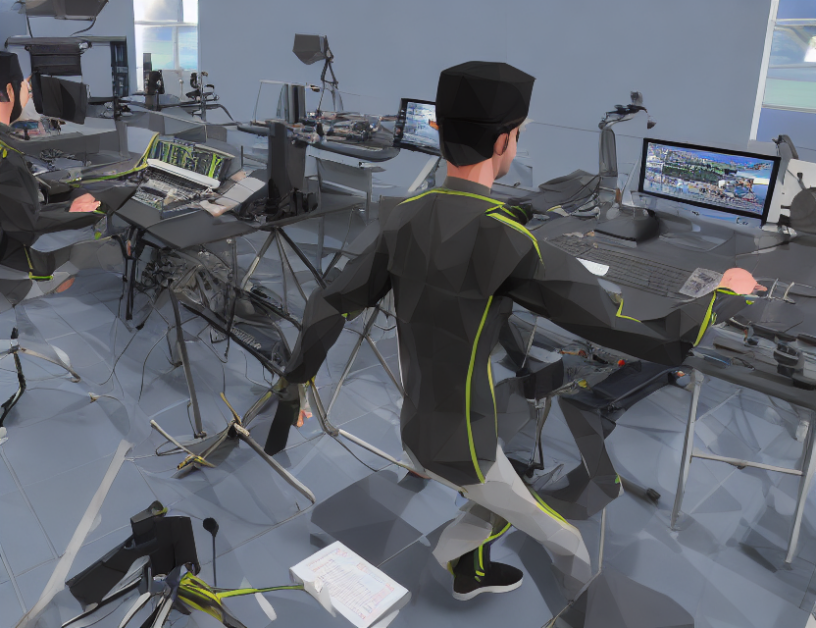