In this article, we explore the impact of various prompting strategies on improving the performance of semantic segmentation models (SAMs). We use a variety of pre-processing techniques, such as scaling, rotation, blurring, and contrast adjustments, to generate diverse images. We then fine-tune SAMs with different amounts of annotated data, ranging from limited to fully annotated datasets. Our findings reveal that combining positive points with bounding boxes or negative points yields the best results, while fine-tuning SAM without bounding boxes leads to worse performance.
To understand how prompting strategies affect SAMs, imagine a student studying for an exam. Just like how different study methods can improve their understanding and retention of information, prompting strategies can enhance the accuracy of semantic segmentation models. By adjusting the pre-processing techniques and fine-tuning with bounding boxes or negative points, we can optimize the performance of SAMs, much like a student tailors their study plan to suit their learning style.
Our results show that fine-tuning SAMs with only positive points or negative points leads to marginal improvements. However, combining both types of points yields significant benefits, demonstrating the importance of balancing positive and negative examples during training. Similarly, adding bounding boxes to the fine-tuning process boosts performance, illustrating how visual context can enhance the accuracy of semantic segmentation models.
In conclusion, our findings demonstrate that prompting strategies play a crucial role in improving the performance of semantic segmentation models. By carefully selecting and combining pre-processing techniques and fine-tuning methods, we can optimize SAMs for better accuracy and robustness. These insights can guide the development and application of SAMs in various computer vision tasks.
Computer Science, Computer Vision and Pattern Recognition
Fine-Tuning SAM for Image Segmentation with Different Prompting Strategies
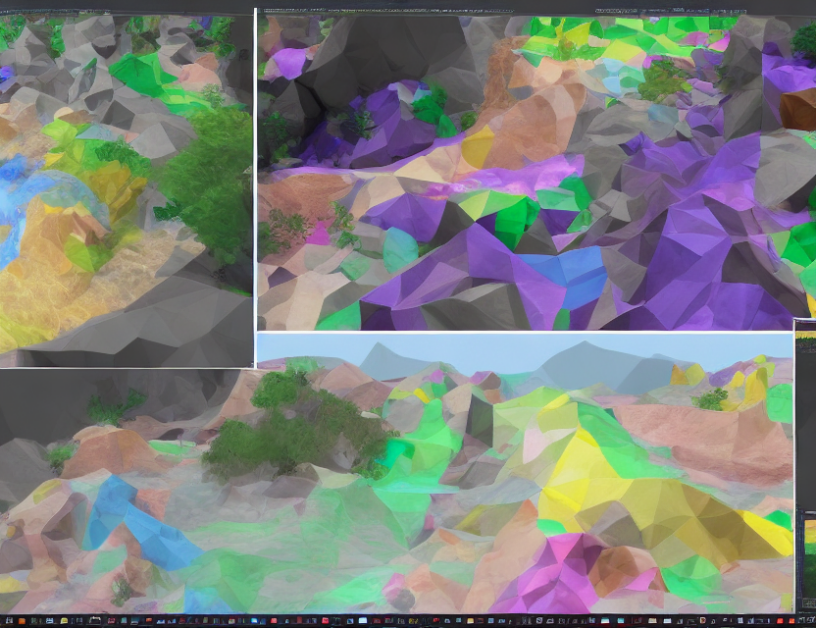