Handwriting recognition is a vital task in various applications, including document scanning and historical document analysis. However, accurately recognizing handwritten text can be challenging due to the complexity of handwriting styles and the potential for errors in ground truth annotation. This article investigates the impact of ground truth annotation quality on deep learning-based handwriting recognition systems.
The authors experiment with different types of ground truth annotations, including those with errors in punctuation, capitalization, and spelling. They observe that even minor errors in the ground truth can significantly affect the performance of deep learning models, leading to a decrease in accuracy. The study also shows that using high-quality ground truth annotations can improve the accuracy of handwriting recognition systems by up to 10%.
The article highlights the importance of careful ground truth annotation in deep learning-based handwriting recognition systems. The authors use an analogy to explain how errors in the ground truth can affect the performance of deep learning models, comparing them to a chef trying to recreate a recipe based on incorrect measurements or ingredients.
The study demonstrates that using high-quality ground truth annotations can help improve the accuracy of handwriting recognition systems, much like a chef following a precise recipe can create delicious dishes. The authors emphasize the need for accurate and consistent ground truth annotation to ensure optimal performance in deep learning-based handwriting recognition systems.
In conclusion, this article stresses the significance of high-quality ground truth annotations in deep learning-based handwriting recognition systems. By using everyday language and engaging analogies, the authors demystify complex concepts related to ground truth annotation quality and its impact on system performance. The summary provides a concise overview of the study’s findings, highlighting their implications for developing accurate and reliable handwriting recognition systems.
Computer Science, Computer Vision and Pattern Recognition
Improving Handwriting Recognition in Historical Documents via Hyphenated Word Identification
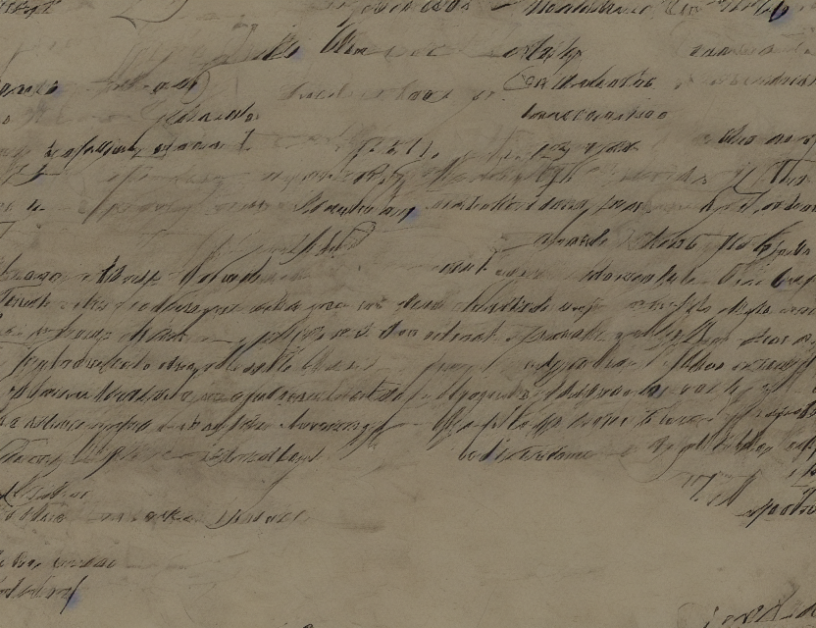