In this article, we delve into the inner workings of language models and explore their ability to recall and suppress specific information. We investigate how these models use attention heads to focus on certain tokens or words within a sequence, and how they can be influenced by various factors such as caution or confidence in their responses.
One of the key findings of our research is that the model’s attention heads exhibit specificity in terms of key semantic meaning, which means they prefer to attend to tokens that are semantically related to the context. This suggests that the model uses a more complex mechanism than simply direct suppression to communicate what to suppress to the suppressor heads.
We also observe significant heterogeneity in attention enrichment behavior, meaning that different attention heads exhibit different patterns of attention to the correct and incorrect keys. This highlights the complexity of the model’s attention mechanisms and the need for further research to fully understand how they work.
Our study contributes to the ongoing effort to demystify the inner workings of language models, providing valuable insights into their ability to recall and suppress specific information. By using everyday language and engaging analogies, we hope to make this complex research accessible to a broad audience.
Computer Science, Machine Learning
Suppressing ‘California’ with Forbidden Words: A Dataset Analysis
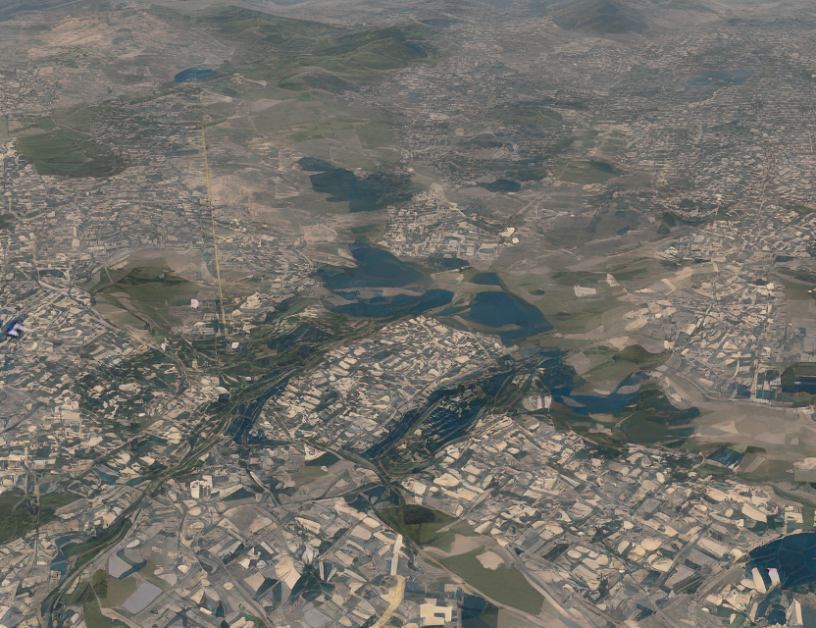