In recent years, there has been a growing interest in novel view synthesis, which involves generating images from different perspectives or angles. This technology has numerous applications, including augmented reality and virtual reality content creation. However, most existing methods rely on time-consuming iterative optimization techniques that can’t generalize well to new images.
To address this limitation, researchers propose Zero123, a novel approach that utilizes a powerful diffusion model to generate high-quality images with good generalization ability and efficiency. Unlike other methods, which require a single source-view image as input, Zero123 can accept any random view as input, making it a promising alternative for novel view synthesis.
To evaluate the effectiveness of Zero123, researchers compare it to two other state-of-the-art methods: Zero123 and Image Variations (IV). Although Neural Radiance Field (NeRF) is widely adopted for novel view synthesis, it only works well when a large number of images are available. Therefore, researchers also compare Zero123 to DietNeRF, a technique that regularizes NeRF using a CLIP image-to-image consistency loss.
The results show that Zero123 outperforms the other methods in terms of both quality and efficiency. It can generate high-quality images with good generalization ability even when only a limited number of images are available. Moreover, it is computationally more efficient than the other methods, making it an attractive choice for real-world applications.
In summary, Zero123 offers a promising alternative for novel view synthesis by utilizing a powerful diffusion model to generate high-quality images with good generalization ability and efficiency. Its ability to accept any random view as input makes it particularly appealing for real-world applications where a limited number of images may be available.
Computer Science, Computer Vision and Pattern Recognition
Unlocking Novel View Synthesis: U-Net Conditioning Dominates CLIP Embedding
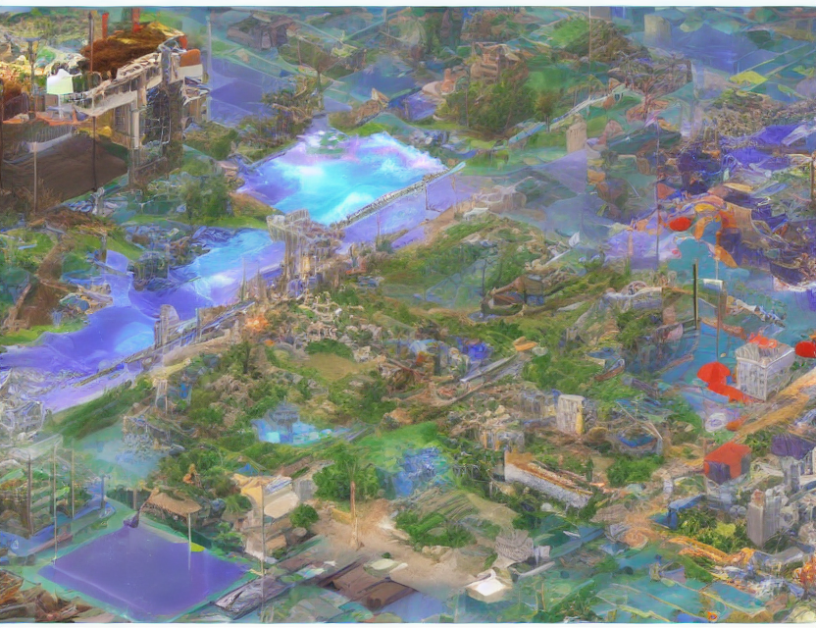