Domain generalization (DG) is a crucial aspect of artificial intelligence, enabling AI models to adapt to unseen domains or environments. In this article, we delve into how leveraging the power of pre-trained language models like CLIP can significantly improve DG in computer vision tasks. The proposed approach, titled CLIP-based Student-Teacher Networks (CSSN), employs a CNN backbone with CLIP features to extract robust representations. The technique harnesses the capabilities of established foundation models like CLIP to advance on DGSS (Domain Generalization with Self-Supervised Training).
To enhance domain randomization, we adopt a text-to-image diffusion model that generates photo-realistic images while being conditioned on textual prompts generated using a Large Language Model. This approach broadens the diversity of generated images used for self-training the model, which in turn strengthens its generalizability across diverse domains.
The predicted pseudo labels are refined with the help of Segment-Anything Model (SAM) in a student-teacher fashion. This collaborative strategy aims to fortify the model’s ability to generalize across different domains. The proposed approach, CSSN, demonstrates promising results on several benchmark datasets, showcasing its potential for tackling challenging DG tasks.
In simple terms, CLIP is like a superhero in the world of AI, providing robust feature representations and image-text alignment capabilities that have been instrumental in various vision applications. By harnessing the power of CLIP, our approach employs a CNN backbone to extract meaningful features and advances on DGSS by leveraging the strengths of established models like CLIP.
Imagine CLIP as a wise old teacher who has mastered the art of feature representation. By sharing their knowledge with younger models, these "teachers" can help improve the newer models’ ability to generalize across different domains. In essence, CSSN is a novel approach that leverages the collective wisdom of established models like CLIP to create more robust AI systems capable of adapting to unseen domains.
Computer Science, Computer Vision and Pattern Recognition
Advancing Domain Generalized Semantic Segmentation with Collaborative Foundation Models
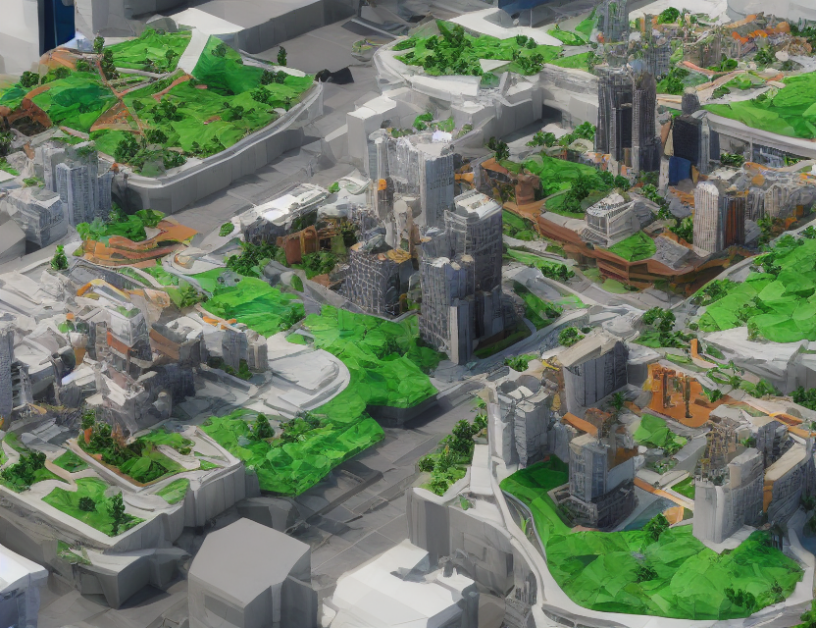