In this research, we propose a novel approach to raw image reconstruction using a text-conditioned diffusion model. Our method leverages captions from the COCO dataset to improve the reconstruction results in low-light conditions. By adding the caption information to the diffusion model, we demonstrate that it contributes significantly to the reconstruction of fine details and textures in the image.
To train our model, we firstly established a pipeline for fine-tuning a pre-trained diffusion model on real-world data captured using a smartphone camera. We then added the caption information to the model and fine-tuned it again. The contributions of our work are twofold: Firstly, we present a novel method for raw image reconstruction that incorporates textual information to improve the quality of the reconstructed image. Secondly, we demonstrate that the additional caption information provides valuable context to the diffusion model, enabling it to better restore fine details and textures in low-light conditions.
Our approach is unique compared to other non-text-conditioned methods, which rely solely on the noise statistics of the scene. By incorporating the caption information, our model can leverage the additional context provided by the photographer to better understand the content of the scene and improve the reconstruction results.
To evaluate our method, we conducted experiments using both synthetic and real-world images. The results show that our text-conditioned diffusion model significantly outperforms non-text-conditioned models in terms of image quality and details restoration.
In summary, our research presents a novel approach to raw image reconstruction that leverages captions from the COCO dataset to improve the quality of reconstructed images in low-light conditions. By incorporating textual information into the diffusion model, we demonstrate that it provides valuable context for better understanding the content of the scene and restoring fine details and textures. Our method has significant potential applications in various fields such as image enhancement, segmentation, and generation.
Computer Science, Computer Vision and Pattern Recognition
Assessing the Significance of Text Contribution in Image Reconstruction under Noisy Conditions
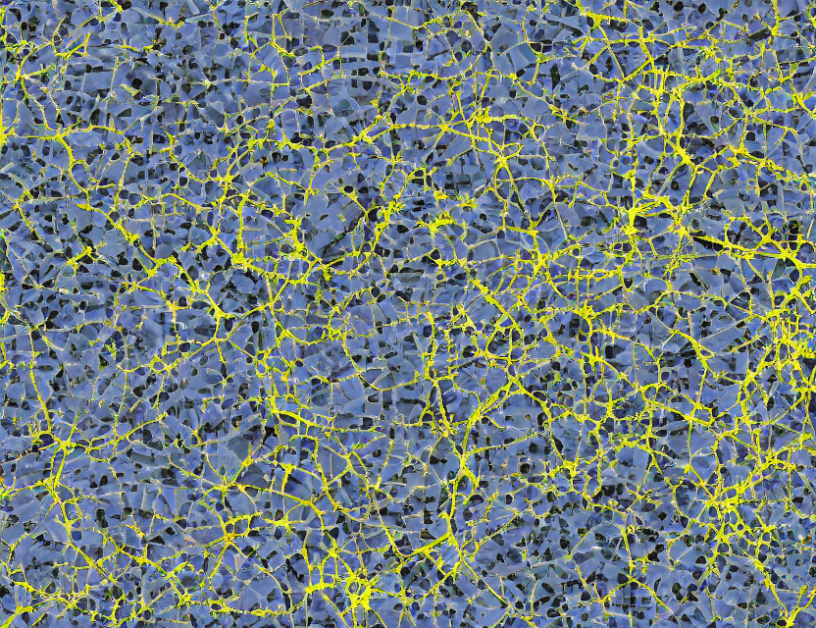