In this paper, Zekang Li, et al. propose a unified multimodal transformer model for audio-visual scene understanding, which is essential for various applications such as video captioning, audio-visual question answering, and dialogue systems. The authors aim to bridge the gap between text and video by leveraging the complementary information from both modalities.
The proposed model, called Multimodal Transformer (MT), combines the strengths of both text and video modalities by utilizing a transformer architecture that processes input sequences from both modalities simultaneously. The MT model consists of two parts: a multimodal encoder that fuses the text and video features, and a decoder that generates the output sequence.
The authors evaluate the performance of the MT model on several benchmark datasets, including DSTC7 at AAAI2019 workshop and the recently introduced Counterfactual VQA (CVQA) dataset. The results demonstrate that the MT model outperforms existing state-of-the-art models in audio-visual scene understanding tasks.
The authors also perform a series of ablation studies to analyze the effectiveness of different components of the MT model. These experiments show that the multimodal encoder is crucial for fusing the information from both modalities, while the decoder plays a critical role in generating coherent and informative output sequences.
Furthermore, the authors demonstrate the versatility of their approach by applying it to various tasks such as video captioning, audio-visual question answering, and dialogue systems. They show that the MT model can be easily adapted to these tasks by modifying the decoder architecture and training the model on task-specific datasets.
In summary, this paper presents a significant advancement in the field of multimodal AI by proposing a unified transformer model that bridges the gap between text and video modalities for audio-visual scene understanding. The proposed MT model demonstrates impressive performance on various tasks and has broad applications in multimedia processing, natural language processing, and human-computer interaction.
Computation and Language, Computer Science
Bridging Text and Video: A Universal Multimodal Transformer for Audio-Visual Scene-Aware Dialogue Generation
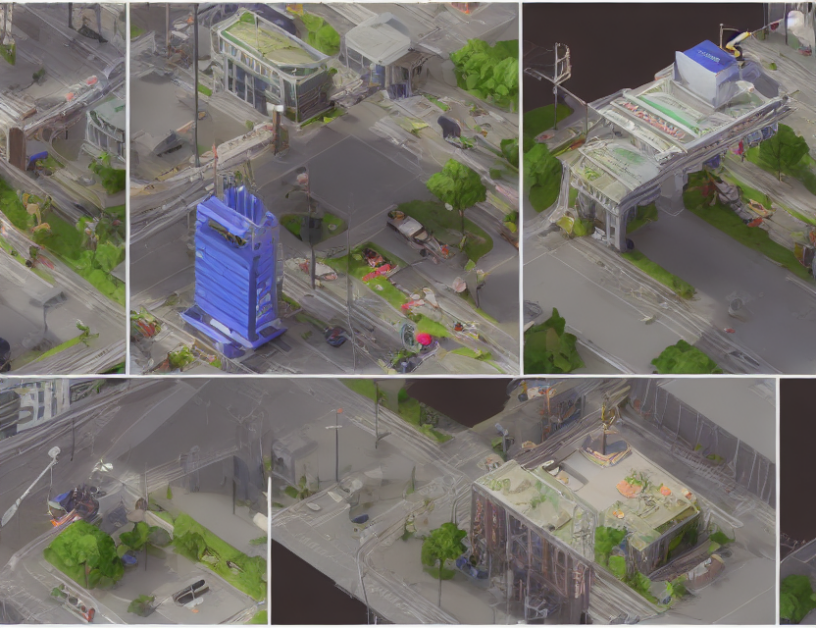