CNC machining is a crucial aspect of modern manufacturing, but it’s labor-intensive and requires expert knowledge. Traditional methods involve crafting intricate sets of instructions for CNC machines to produce complex products with precision. However, these methods are slow and inefficient, limiting the industry’s ability to adapt to changing demands. To address these challenges, researchers have developed a deep learning-based approach that automates CNC machining.
The proposed method leverages self-supervised learning techniques to train a neural network on a vast dataset of 3D models. The network learns to generate the essential operation parameters required for constructing the desired object. This process is faster and more accurate than manual programming, making it an excellent alternative to traditional CNC machining methods.
The authors evaluate their approach using various quantitative and qualitative experiments on ABC and ShapeNet datasets. They compare their reconstruction performance with state-of-the-art 3D CAD reconstruction methods, including CSG-Stump, ExtrudeNet, CAPRI-Net, and SECAD-Net. The results show that their method outperforms these existing approaches in terms of accuracy and efficiency, demonstrating its potential to transform manufacturing.
The proposed approach has several advantages, including faster adaptation to new tasks, reduced labor requirements, and improved precision. However, the authors acknowledge that refining their method to select the most appropriate operation type at each step is crucial for optimal performance. They plan to explore different tool shapes and operations in future research, broadening the range of machining applications.
In summary, deep learning-based automation has the potential to revolutionize CNC machining by improving efficiency, reducing costs, and increasing precision. By leveraging self-supervised learning techniques, researchers have developed a transformative approach that can adapt to changing manufacturing demands. As the field continues to evolve, we can expect even more innovative solutions to emerge, further transforming modern manufacturing.
Computer Science, Computer Vision and Pattern Recognition
Deep Learning for Efficient and Precise 3D Object Manufacturing
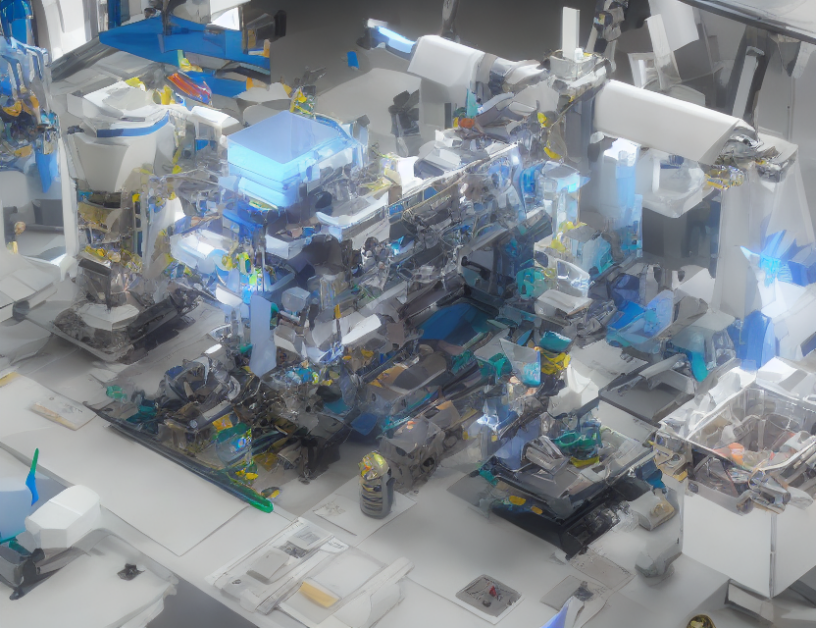