In this article, the authors explore the concept of cross-attention modulation in image editing, focusing on its limitations and potential solutions. They begin by explaining that previous studies have shown that attention maps with a resolution of 16 × 16 capture the most detailed semantic information, but these maps can be noisy and require additional processing to extract meaningful features.
The authors introduce the Disentangle Sample method as a solution to this problem. This technique separates the editing areas from irrelevant regions by applying a Gaussian filter to the cross-attention map. The authors demonstrate that this approach effectively improves the editing ability, especially in cases where fine details are required.
However, the article also acknowledges certain limitations of the proposed method. For instance, smaller attention maps may restrict the ultra-fine editing ability to some extent. Moreover, the effectiveness of the Disentangle Sample method relies heavily on the capabilities of the pretrained IP2P model used in the study.
To better understand these concepts, consider an analogy with cooking. Imagine that attention maps are like a recipe book, containing various instructions for preparing dishes. The resolution of these maps represents the level of detail in each instruction, similar to how a higher number of ingredients can result in more detailed and complex recipes.
Just as it’s important to filter out unwanted ingredients when cooking, the Disentangle Sample method helps remove irrelevant areas in attention maps, allowing for more precise editing. However, just as a recipe book may have limitations based on the ingredients available, the effectiveness of this approach depends on the quality and capabilities of the pretrained model used.
In summary, the article provides insights into the challenges of cross-attention modulation in image editing and proposes a solution to overcome these limitations. By using the Disentangle Sample method, editors can enhance their ability to create detailed and accurate images while avoiding unwanted areas. Although this approach has its own limitations, it represents an important step towards improving the accuracy and efficiency of image editing techniques.
Computer Science, Computer Vision and Pattern Recognition
Disentangling Image Manipulation: Modulating Attention for Precise Editing
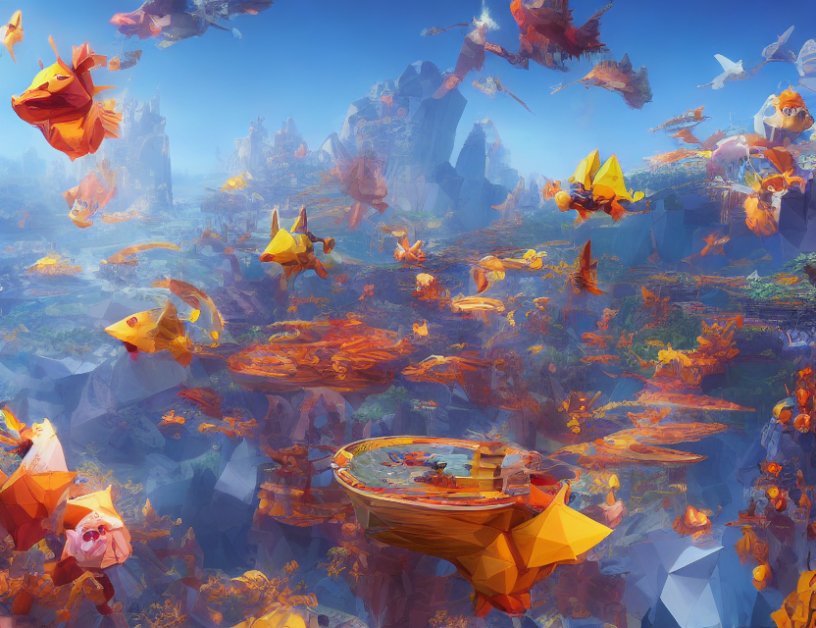