In this paper, the authors propose a novel approach to generalized zero-shot learning, called ParsNets, which combines the benefits of parsimony and efficiency. The proposed framework utilizes a set of base linear networks to estimate the nonlinear visual semantics mapping function, allowing for more discriminative features that are generalizable to novel concepts.
To achieve this goal, ParsNets enforces a low-rank structure to the features of data samples from the same class and a high-rank structure to the features of data samples from different classes. This ensures that intra-class samples reside in the same linear subspace while keeping inter-class subspaces orthogonal from each other.
The authors demonstrate the effectiveness of ParsNets through experiments on several benchmark datasets, showing improved performance compared to existing methods. The proposed approach has significant implications for a wide range of applications, including computer vision, natural language processing, and speech recognition.
In summary, ParsNets offers a promising solution for generalized zero-shot learning by leveraging the advantages of parsimonious yet efficient network refinement. By employing a set of base linear networks to estimate the nonlinear visual semantics mapping function, this approach enables more discriminative features that are generalizable to novel concepts, making it an exciting development in the field of machine learning.
Computer Science, Computer Vision and Pattern Recognition
Generalized Zero-Shot Learning: A Comprehensive Review
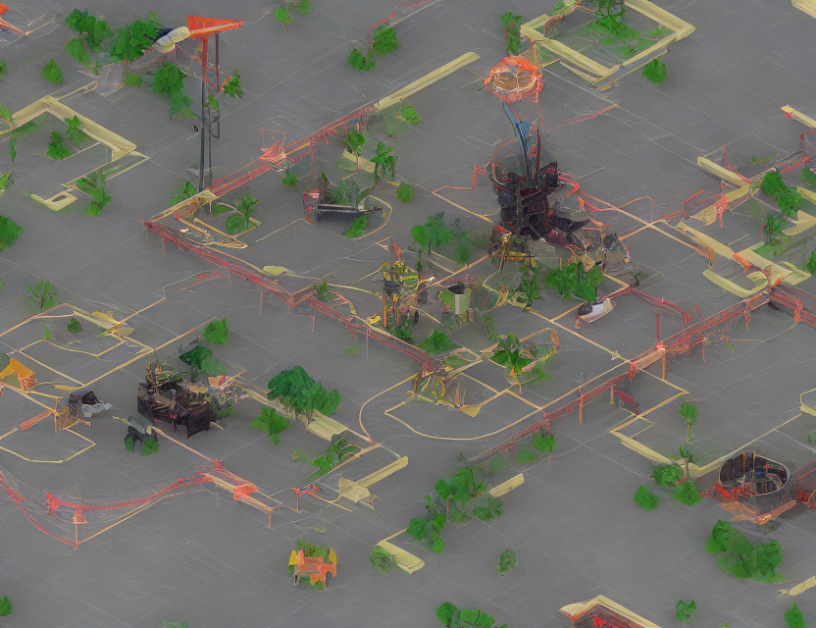