The article begins by discussing the challenges of multi-modal object-entity relation extraction and the limitations of traditional approaches that rely solely on textual or visual information. The authors then introduce the MORE framework, which combines both modalities to improve accuracy and robustness. The proposed method consists of three stages: data construction, dataset analysis, and attribute-aware textual encoder.
In the data construction stage, the authors collected multimodal news data from The New York Times and Yahoo News between 2019 and 2022, resulting in a candidate set of 15,000 instances covering various topics. They then filtered out unqualified data and obtained a meticulously selected dataset for their research purposes.
In the dataset analysis section, the authors provide a detailed comparison of MORE with other existing datasets for relation extraction, demonstrating the unique features and advantages of their approach. They also discuss the importance of modeling attribute-aware relationships between objects in the textual encoder stage, which enhances the accuracy of object detection and connection prediction.
The authors conclude by highlighting the potential applications of MORE in various fields, such as image and video analysis, natural language processing, and cognitive computing. They also suggest future directions for research, including incorporating additional modalities and improving the efficiency of the proposed method.
In summary, MORE is a groundbreaking approach to multi-modal object-entity relation extraction that leverages both textual and visual features to improve accuracy and robustness. By combining these modalities, MORE can identify objects and their connections more effectively, making it a valuable tool for various applications in computer science and related fields.
Multimodal Object-Entity Relation Extraction: An Analysis of MORE Dataset
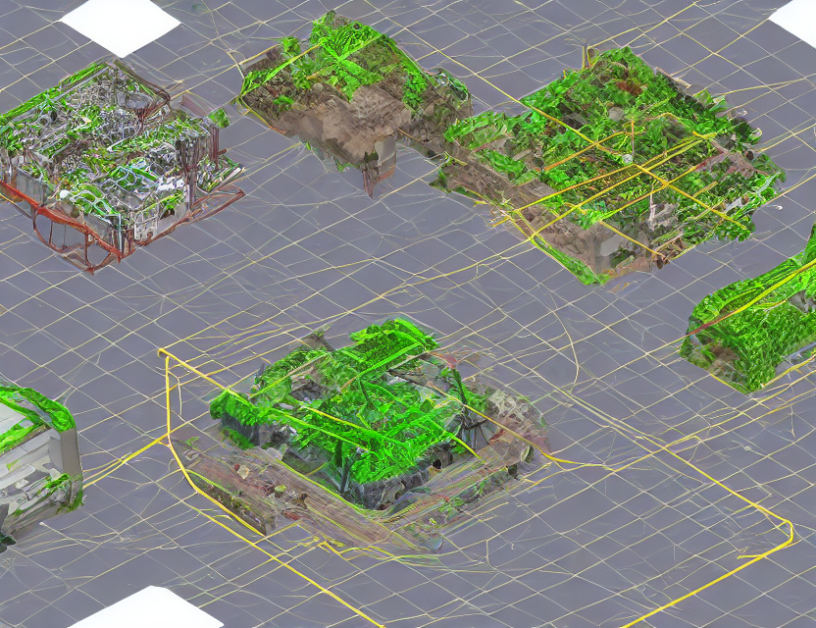